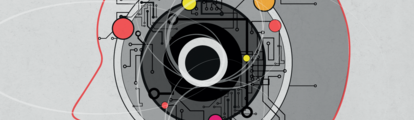
The Ethics of AI
Four deep learning experts discuss the ethical implications of using advanced technologies in eye care – and potential solutions to the problems they present
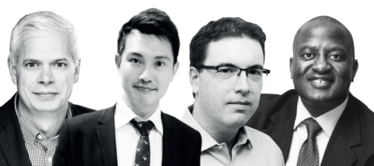
Michael D. Abramoff is a retina specialist, physician/scientist, the Robert C. Watzke, Professor of Ophthalmology and Visual Sciences, of Electrical and Computer, and Biomedical Engineering at the University of Iowa, and Founder and Executive Chairman of IDx. He is based in Iowa City, Iowa, USA.
Pearse Keane is an NIHR Clinician Scientist at the UCL Institute of Ophthalmology, and Consultant Ophthalmologist at Moorfields Eye Hospital NHS Foundation Trust in London, UK.
Stephen Odaibo is a retina specialist, computer scientist, mathematician, full-stack AI engineer and co-founder of RETINA-AI in Texas, USA.
Daniel Ting is a Vitreoretinal Specialist at the Singapore National Eye Center, Assistant Professor of Ophthalmology at Duke-NUS Medical School, Singapore, and Adjunct Professor at the State Key Laboratory of Ophthalmology at Zhongshan Ophthalmic Center in China.
The first part of the AI discussion can be found here: theophthalmologist.com/subspecialties/ophthalmic-frontiers-ai
Is AI and deep learning being developed predominantly in and for industrialized countries?
Abramoff: Though there are many advantages to low-cost, autonomous AI solutions that could work well in the developing world – including improved access and better quality of care – the public fear it. We saw a similar reaction to gene therapy when it first came out. Unfortunately, there was a moratorium on the subject for many years after a number of people died in poorly designed studies. It was only very recently that gene therapy was FDA-approved in retina (for LCA). These backlashes have real consequences – when there is a moratorium, there is no funding for research, and institutions close. Theranox was another problem – again, inappropriate unethical behavior destroying a lot of trust in quality in healthcare – which is why we need to do it right. Start with the highest standards, which in the United States, is the FDA, and then expand to other countries where there may be less regulatory oversight.
Keane: There are huge barriers for the implementation of some of these technologies, but at the same time, there are also lots of opportunities. What I think you’ll see is a confluence of improved telecommunications. As we start to see the rollout of 5G, for example, we will see increasing use of cloud-based infrastructure there may offer advantages to low and middle-income countries – though a lack of legacy infrastructure has to be taken into consideration. It might be the case that some of these countries can leapfrog more developed areas and start to do exciting things with these technologies.
One of the things I’m particularly interested in is automated deep learning systems. My team recently published a paper showing how healthcare professionals with no coding experience were able to train AI systems using deep-learning platforms. While those systems can’t be used for direct patient care (in part because they would have to go through a robust regulatory process), you could imagine that this approach would allow the proliferation of novel clinical research applications for AI in both low and middle-income countries, and the developed world.
Odaibo: I have a privileged view into this being a dual citizen, both American and Nigerian, and being active in both countries. Developing countries have some notable advantages in terms of data, costs, readiness and willingness to implement AI solutions. Industrialized economies also clearly have advantages, such as well-developed regulatory systems, infrastructure, and established data systems. Each advantage can potentially resemble or be a disadvantage and vice versa. For instance, the absence of existing electronic medical records systems in developing countries provides an opportunity to “leapfrog” by starting out with AI-ready next generation EMR systems that are truly-continuous learners, and are unencumbered by impeding static legacy systems. On the other hand, the relative dearth of existing electronic data does mean starting nearly from scratch for a lot of developing countries.
Ting: The ethical dilemma of applying AI in healthcare is widely debated. For developing countries, I see a huge potential role for AI to accelerate the standard of care, especially in countries with a shortage of eye specialists. At present, most deep learning systems are found in developed countries, which is unsurprising, given the availability of the technical expertise and clinical datasets. In 2019, my group collaborated with Zambian and UK ophthalmologists to report on the feasibility of using deep learning to screen for diabetic retinopathy in the African population. The findings were published in the Lancet Digital Health (Bellemo et al, 2019). Of course, the question is then whether the local community has the capacity and expertise to treat those who require treatment. These are some of the medical and ethical questions one may need to ask. If there is an insufficient number of doctors to treat a particular disease, is there a role to screen for a particular disease in the specific population? To clinically deploy an AI algorithm, it is always important to build a robust ecosystem surrounding it. This ecosystem requires medical, technical and financial support from multiple stakeholders.
Are certain ethnicities “favored” when algorithms are created, while some are left behind?
Abramoff: There are legitimate concerns about racial and ethnic bias in AI. It is important that autonomous AI is designed and validated in a way that ensures those concerns are addressed, and any inappropriate bias is corrected. As with everything, transparency is paramount.
Odaibo: Yes, there has been legitimate concern around the issue of equality in development of algorithms. One is that AI is only as good as the data it is fed, and naturally emulates whatever biases are encoded in that data. More specifically, the big concern in countries such as the US and the UK is that much of the existing data was annotated to favor Caucasians and to favor men, and in some cases to explicitly disfavor other ethnic groups and women. To have truly generalizable algorithms that are fair, the distribution of training data has to be representative of the population, and, furthermore, has to be explicitly engineered to not be biased – because representative but biased data will yield a biased algorithm.
Ting: The AI algorithms are often created in the developed countries such as US/Europe that are predominantly Caucasian, whereas in Asia (like Singapore), we have a multi-ethnic population consisting of people of Chinese, Malay and Indian origin. Given that the AI algorithm may be developed using certain populations, it is always important to test the generalizability of the algorithm to other populations.
What happens when the algorithms get something wrong? Who would be deemed responsible: producers, coders, or practitioners?
Abramoff: In autonomous artificial intelligence, the computer makes a clinical decision without any human oversight. Since the system makes a decision the way a doctor would, the manufacturer of said system should assume medical liability – the way a doctor would. It is a question of legal liability. It took about eight years of discussion with the FDA to work out a way to validate the safety, efficacy and equity of my own autonomous AI company, IDx, starting in 2010. It is therefore good to see that the American Medical Association included liability as a requirement for autonomous AI in its AI Policy document that came out last year.
Keane: It depends if you’re talking about an autonomous system or a decision-support system. If it is an autonomous system, then medical liability will be with the manufacturer of the AI system. If it is a decision-support system, it is the doctor who makes the final decision, which complicates things. In the US, if a doctor treats a patient according to the current standard of care, then they are usually protected against claims of medical malpractice – but if they do something that goes against the standard of care, then they may assume some legal liability.
The whole promise of AI is that it allows us to personalise our treatment of individual patients beyond the standard of care. As a doctor, you can be put in a tricky position. You have a patient, and you know the standard of care is X, but the AI system is telling you to do Y. If you do what the AI systems tells you and the patient reacts badly, you could be in a difficult situation legally. On the other hand, suppose that same doctor decides to go with the standard of care, despite the AI system telling them otherwise. If things go wrong for the patient, they could sue the doctor because the AI system told them to do Y, and they did X. In both scenarios, the doctor could be legally liable. It is a very difficult situation and, ultimately, one that professional bodies need to advise on. Guidelines would offer reassurance to ophthalmologists and patients that physicians are doing the right thing when faced with these choices.
Odaibo: Coders – and I say that as a coder. When we deploy fully autonomous systems – and we are essentially there in diabetic retinopathy for instance – it would make sense for AI companies to assume the responsibility that physicians currently assume. In a nutshell, AI companies will need malpractice insurance.
Ting: This is a question that I don’t think we have a good answer to at the moment. In my personal opinion, I think the practitioners should ultimately have the responsibility to override the system if the wrong diagnosis is given by the AI software. Many newspaper headlines and research have reported that AI can perform better than humans, and in some cases that may be true. Nevertheless, in the healthcare setting, I always think that physicians should be the gatekeepers who assess and utilize all the different health technologies to improve patients’ care. The clinical decision should be made based on the patient’s clinical presentation, examinations and investigations. AI should always be treated as an assistive tool, instead of the sole decision-making tool for medical diagnoses. Furthermore, prior to adoption of any AI software, the physicians should also carefully appraise all the evidence that are available in the literature, the same way we assess any new drug released into the market.
If AI is used for analysing results/images, is it likely to increase the number of referrals? Are the world’s healthcare systems prepared for such an increase?
Keane: AI is not magic. While it has the potential to be a powerful tool, it cannot solve complex diseases in isolation. It will only ever work in the context of good patient pathways and widespread adoption by healthcare professionals, and even in situations where you’ve got a good pathway, there could be other social, cultural or public health barriers to its success. I think it is important that we take a nuanced view of this.
Odaibo: It will most certainly increase the number of referrals, but I believe it is good to know who out there needs care, and what the stage of their disease is, regardless of the capacity to address it. The first step to solving a problem is knowing about the problem.
Ting: I think the application of AI is likely to increase the number of referrals to tertiary eye care settings. Hopefully these will not be false positive referrals, although this could also happen if the operating threshold of the AI algorithms are not set properly. Some healthcare systems may not be ready to cope with this, especially in those countries with long waiting times within their healthcare systems.
What regulations exist already for implementing AI/deep learning around the world, and what still needs to be done in this area?
Abramoff: In the USA, the FDA and the Federal Trades Commission (FTC) have worked for years to create appropriate standards. Safety and efficacy have to be evaluated, as should liability for deviations from the performance, before AI is integrated into the larger healthcare system.
Doctors are not typically validated against outcomes – AI systems are. Therefore, studies should not compare AI to doctors’ performance, but to patient outcomes and surrogate outcomes. That is important, as many AI systems are still being compared to a doctor, and we do not know how well that corresponds with outcomes. We do our own studies, and self-regulate, but it’s good to have an independent body to oversee this, such as the FDA, which is very rigorous in its demands.
Keane: Systems certainly cannot be used on patients until they’ve received regulatory approvals from the appropriate regulator. The way AI systems will be regulated is likely to evolve in the coming years, as the FDA and others learn about the complexities – especially the issue of dealing with systems that learn “on the job” or have the potential to change their accuracy as they’re exposed to more data. Currently algorithms are trained with data, but then they’re locked in place, so they’re not changing performance in real life. However, in the future algorithms could change over time – although that’s currently very far from being a reality in the healthcare setting.
It is important to combine our excitement and enthusiasm for this new technology with caution and realism that it won’t automatically cure everything. Before we even start to talk about referrals, we have to make sure that these algorithms are robustly, clinically validated because although they have great potential, there are a lot of ways in which they could go wrong. We need to bring the same level of rigour to the validation of algorithms as we would to a new drug or surgical procedure.
Odaibo: Much work is needed in the area of appropriate regulation that helps and improves the health of the public. The biggest barrier is knowledge, as this is a highly interdisciplinary area that does require some level of algorithmic understanding on the part of regulators. There is clearly a knowledge gap around the world in this area, including in the most developed systems, such as the FDA. However, good efforts are underway to close this gap via frequent communication and community engagement.
For instance, in June 2019, I along with a number of other healthcare AI experts helped draft the Alliance for AI in Healthcare’s feedback to the FDA. This FDA-initiated feedback request was regarding a draft of its Regulatory Framework for AI Software as a Medical device (1). Such efforts demonstrate a sincere desire by the FDA to understand all facets of this important emerging field.
Ting: At present, the FDA has already published a guideline earlier this year to treat AI algorithms as medical devices. In the guideline, it states that the AI algorithm will need to be submitted and appraised based on what the intended use. The WHO has also published recommendations on digital interventions for health system strengthening. The STARD guideline has always been the method we use to report a new diagnostic device, whereas the CONSORT is used for reporting clinical trials results. Nevertheless, it will be important to create a new AI reporting guidelines to take into account the clinical settings in which the algorithm will be used, to increase the success of technology transfer from bench to bedside.
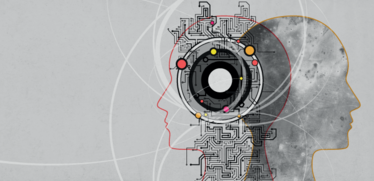
- AAIH, “Proposed Regulatory Framework for Modifications to Artificial Intelligence/Machine Learning (AI/ML)-Based Software as a Medical Device (SaMD) - Discussion Paper and Request for Feedback. — Comments from Alliance for AI in Healthcare”, Available at: https://bit.ly/3ausc7t.
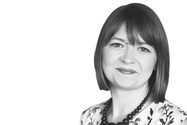
Having edited several technical publications over the last decade, I crossed paths with quite a few of Texere's current team members, and I only ever heard them sing the company's praises. When an opportunity arose to join Texere, I jumped at the chance! With a background in literature, I love the company's ethos of producing genuinely engaging content, and the fact that it is so well received by our readers makes it even more rewarding.