
Ophthalmic Frontiers: AI
Four AI and deep learning gurus discuss the use of these advanced technologies in eye care – and predict where they may take us next
Michael D. Abramoff, Pearse Keane, Stephen Odaibo, Daniel Ting | | Longer Read
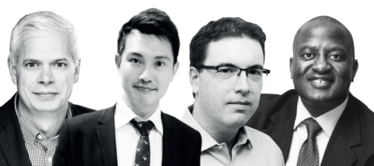
From left: Michael D. Abramoff, Daniel Ting, Pearse Keane and Stephen Odaibo.
Meet the AI Gurus
Michael D. Abramoff is a retina specialist, physician/scientist, the Robert C. Watzke, Professor of Ophthalmology and Visual Sciences, of Electrical and Computer, and Biomedical Engineering at the University of Iowa, and Founder and Executive Chairman of IDx. He is based in Iowa City, Iowa, USA.
Pearse Keane is an NIHR Clinician Scientist at the UCL Institute of Ophthalmology, and Consultant Ophthalmologist at Moorfields Eye Hospital NHS Foundation Trust in London, UK.
Stephen Odaibo is a retina specialist, computer scientist, mathematician, full-stack AI engineer and co-founder of RETINA-AI in Texas, USA.
Daniel Ting is a Vitreoretinal Specialist at the Singapore National Eye Center, Assistant Professor of Ophthalmology at Duke-NUS Medical School, Singapore, and Adjunct Professor at the State Key Laboratory of Ophthalmology at Zhongshan Ophthalmic Center in China.
What is your experience with artificial intelligence (AI) and deep learning in ophthalmology?
Abramoff: I continue to see patients as a fellowship trained retina specialist, and for decades studied the use of neural networks and artificial intelligence to diagnose and treat diseases. I founded IDx, which created the first ever autonomous artificial intelligence cleared by FDA through the first clinical trial for AI. To do autonomous AI “the right way,” I had to create ethical principles for autonomous AI, that have now led to regulatory and payment pathways for autonomous AI.
I’ve been studying neural networks since 1988 and it was the subject of my Master’s and PhD theses. Since then, I’ve published over 270 peer reviewed journal papers (h-index 61) on artificial intelligence and retinal diseases that have been cited over 30,000 times, as well as many book chapters. I’m also the inventor on 17 US patents and 5 patent applications on autonomous AI algorithms and devices.
My focus is on autonomous AI: AI that makes clinical decisions by itself without human or physician interpretation, because to me that is the only way to decrease cost, improve access, and improve quality in healthcare. In 2018, we completed the first pre-registered, prospective clinical trial, testing the safety, efficacy, and equity of an autonomous AI in real-world clinical care (1).
Keane: My clinical practice is focused on retinal diseases, in particular macular degeneration and diabetic retinopathy (DR). My research at the UCL Institute of Ophthalmology is based on ophthalmic imaging, in particular OCT, and is increasingly focused on applying AI to ophthalmic imaging. When I say “AI,” I’m referring to deep learning, which is a new flavor of AI, responsible for the hype we’ve seen in the past few years. The main reason I’m known in this field is because I initiated a collaboration between Moorfields Eye Hospital and a company called DeepMind in 2015. We formalized it in 2016, and in September 2018 we published the first paper in Nature Medicine, presenting a deep learning algorithm that was able to look at OCT scans, triage them, and diagnose patients, assessing more than 50 different retinal diseases, with performance on a par with world-leading retina specialists.
We are now working with Google Health to implement that as a product that can be used to treat – we hope – millions of people around the world. A lot of my initial AI work began with that industry collaboration, but as a result of my experience over the last few years, I have developed my own expertise in AI within Moorfields.
Odaibo: I am a fellowship-trained retina specialist, as well as a mathematician, computer scientist and hands-on full-stack AI engineer. I spend approximately 20 percent of my professional time on direct patient clinical care, and the remaining 80 percent on AI research and development – especially AI engineering. I single-handedly built the world’s first mobile AI app for eye care providers, and subsequently founded a company, RETINA-AI Health, where a talented team continues to innovate and develop new technologies in this space.
I have been involved with what is now known as “AI” for most of my life, and I obtained advanced degrees in both mathematics and computer science. Anticipating the need for faster computers – quantum computers – to solve optimization problems such as those in machine learning, I have done work in quantum mechanics and published a technical book on the subject while in residency. I have also published a book on finite group theory, the mathematical theory which lays the foundation for quantum concepts. Most recently, my efforts are focused on the practical engineering side of AI for healthcare, as well as on the deployment of AI in various environments. My primary goal now is to improve healthcare access both in the developed and the developing worlds. For instance, we will be presenting our experience on deploying AI for diabetic retinopathy screening in Africa at this year’s Neural Information Processing Systems (NeurIPS) conference in Vancouver – the primary and largest machine-learning research conference in the world.
Ting: To date, my AI lab has published more than 30 AI articles in major journals such as JAMA (2), Nature Medicine (3), Nature Digital Medicine (4), Nature Biomedical Engineering (5) and Lancet Digital Health (6). This could not be done without my mentor, Tien Wong, who has laid out a great platform for me. As the clinical lead for the AI program, I have also received approximately $1.5 million as principal investigator and $25 million as co-investigator/collaborator in research funding. Together with other AI experts, we have led two major AI article reviews (7, 8). I also serve on four editorials boards, where I manage AI and big data-related manuscripts. Even more excitingly, I am also a founding member of the American Academy of Ophthalmology (AAO) AI taskforce committee, which began in October 2019.
AI/deep learning 101
Keane: Deep learning is just a rebranding of artificial neural networks – a concept that has been around since the 1950s. They are computational models that superficially resemble the brain in the way that they process information. They are not pre-designed or pre-specified. You feed them information, such as a digital photo, consisting of a matrix of numbers, which are fed into the layers of a neural network, and you train the neural network to recognize specific examples by repeating the process millions of times.
This approach is proven to be much more powerful than classical computer programming approaches, where you would essentially try to describe the features of a particular phenomenon by using thousands of lines of code.
If you have a million photos of patients with diabetes, some of whom have DR, while others don’t, you can train an algorithm to be able to detect it and decide whether a referral is needed. The Moorfields-DeepMind collaboration has applied this concept to OCT scans: we’ve trained the neural network to be able to look at scans of different retinal conditions divide cases into referrals of different urgency; the key application is determining how quickly a patient should get in front of a retina specialist. It is much more complicated to train neural networks to analyze a three-dimensional OCT scan than it is to deal with a two-dimensional retinal photograph, and this is the aspect of our work we’re most proud about.
Odaibo: AI/Deep Learning in a nutshell is a way to learn from one’s mistakes. The core instrument at the center of almost all AI algorithms is a neural network. Neural networks transform data successively via a network structure into an output that can be compared with the correct label of, say, the disease depicted in an ophthalmic image. If the neural network’s output is different from the correct diagnosis, the neural network is penalized. In the next round of training, it responds to that penalty by attempting to improve its guessing ability. This process continues iteratively until the neural network is able to gradually get the correct answers most of the time. The desirable, but not guaranteed, outcome of training a neural network is called convergence.
One can think of AI as consisting of data science and data engineering. Within this framework, knowledge – such as of disease features – is stored in trained neural network models, housed in a computing architecture that allows for deployment of the AI service for use by people such as physicians and, ultimately, patients. The training of the neural network models is “data science,” while the construction of architecture to house and serve the trained model is “data engineering.”
Ting: AI was first conceptualized in 1956 after a workshop at Dartmouth College. The term “machine learning” (ML) was subsequently coined by Arthur Samuel in 1959 and stated that “the computer should have the ability to learn using various statistical techniques, without being explicitly programmed” (7). Using ML, the algorithm can learn and make predictions based on the data that has been fed into the training phase, using either a supervised or unsupervised approach. ML has been widely adopted in applications such as computer vision and predictive analytics using complex mathematical models. With the advent of graphic processing units (GPUs), advances in mathematical models, the availability of big datasets, and low-cost sensors, deep learning (DL) techniques have sparked tremendous interest and been applied in many industries. DL uses multiple processing layers to learn representation of data with multiple levels of abstraction. DL approaches use complete images, and associate the entire image with a diagnostic output, thereby eliminating the use of “hand-engineered” image features. With improved performance, DL is now widely adopted in image recognition, speech recognition and natural language processing (10).
To build a robust DL system, it is important to have two main components – the “brain” (technical networks – Convolutional Neural Network; CNN) and the “dictionary” or datasets (11). A CNN is a deep neural network consisting of a cascade of processing layers that resemble the biological processes of the animal visual cortex. It transforms the input volume into an output volume via a differentiable function. Inspired by Hubel and Weisel, each neuron in the visual cortex will respond to the stimulus that is specific to a region within an image, similar to how the brain neuron would respond to the visual stimuli, which will activate a particular region of the visual space, known as the receptive field. These receptive fields are tied together to cover the entire visual field. Two classes of cells are found in this region – simple and complex cells.
Broadly, the CNN can be divided into the input, hidden (also known as feature-extraction layers) and output layers. The hidden layers usually consist of convolutional, pooling, fully connected and normalization layers, and the number of hidden layers will differ for different CNNs. The training and development phase is usually split into training, validation, and testing datasets (see below for descriptions). These datasets must not intersect; an image that is in one of the datasets (like training) must not be used in any of the other datasets (like validation). Ideally, this non-intersection should extend to patients. The general class distribution for the targeted condition should be maintained in all these datasets.
Training dataset: Training of deep neural nets is generally done in batches (subsets) randomly sampled from the training dataset. The training dataset is what is used for optimizing the network weights via backpropagation.
Validation dataset: Validation is used for parameter selection and tuning, and is customarily also used to implement stopping conditions for training.
Testing dataset: Finally, the reported performance of the AI algorithm should be computed exclusively using the selected optimized model weights on the testing datasets. It is important to test the AI system using independent datasets, captured using different devices, population and clinical settings. This process ensures the generalizability of the system in the clinical settings.
Abramoff: The problem with building autonomous AI using CNN in the manner described by others is that no one understands how such an AI makes its clinical decision. Because the CNN’s performance depends entirely on the training data, and not on any understanding of the disease markers, it is susceptible to catastrophic failure, as we and other have shown, as well as to racial and ethnic bias (9).
Therefore, at IDx we use CNNs and other AI algorithms in a very different manner, building AI using many detectors, each of which detect the markers of disease themselves, which are invariant to race, ethnicity, and age, and combine their outputs to a patient-level clinical output. It is this focus on designing autonomous AI – so it is maximally reducible to characteristics aligned with scientific knowledge of human clinician cognition – that seems to have made regulators and physicians more comfortable with autonomous AI.

Which unmet needs in ophthalmology can be met by AI – and how?
Keane: In the UK, ophthalmology has the highest number of out-patient appointments: nearly 10 percent of all NHS clinic appointments, and patient numbers are rising rapidly.
Globally, there is an aging population with increased prevalence of diseases like diabetes: 25 percent of patients over the age of 60 in the EU have early or intermediate AMD (12). As the world’s population gets older, it becomes more than just a concern about retinal diseases – it’s a concern that is inherent in the aging process itself. If you could imagine tools like AI being used in every person over 60 in every country in the world on a regular basis, as part of an assessment of aging populations and general health, the scope is huge. If we don’t come up with new and innovative approaches, we’re going to be in trouble. It’s not a question of luxury, there’s an urgency to come up with innovative solutions.
Odaibo: AI has rightly been compared with electricity in the sense that it will permeate and find applications in essentially all areas of human endeavor, including ophthalmology. The more obvious applications include image classification, like using AI to tell whether a fundus photograph depicts moderate DR or mild DR. Most such image classifications have not yet been done, but they could exist in the future for conditions such as corneal dystrophies or retinal dystrophies. Non-image based ophthalmic disease classification is an area that is essentially untouched so far; there, the input is not an image, but genetic, demographic, metabolomic, symptomatologic data, and some combination of those. Another broad area of application, and one in which RETINA-AI has begun work, is in the construction of generative models for latent feature exploration and synthetic data generation. There is a myriad of opportunities there for personalized pharmaceutical development and synthetic data generation.
Ting: By 2050, the world’s population aged 60 years and older is estimated to be 2 billion – up from 900 million in 2015 – with 80 percent living in low- and middle-income countries. People are living longer, and the pace of aging is faster than ever. Because of this, there is a need for long-term surveillance of many ocular and systemic conditions like DR, glaucoma, AMD, and cardiovascular conditions. Population expansion also creates pressure to screen for important causes of childhood blindness, such as retinopathy of prematurity (ROP), refractive error, and amblyopia. For example, diabetic patients require lifelong screening for DR. With countries in Africa having just one doctor per 300,000 people, this is simply not possible. Thus, AI could significantly improve the screening rate – although the deployment of such AI technology requires careful planning, infrastructure and specialists’ support for those with vision-threatening DR.
What are the main barriers to clinical adoption of AI?
Abramoff: Building an AI algorithm is now relatively easy, and as I like to say, diagnostic AIs are a commodity. What is challenging is building AI into a system, aligned with clinical guidelines, and clinical decision making, robust and easy to use by existing staff in the clinic, in order to move specialty care to primary care, or into the community. Rather than having to travel to a retina specialist, you can now go to a clinic at a grocery store to get a diabetic retinal screening. Minimal training in taking good-quality images and operating a robotic camera, and integration into the healthcare system – this is what really counts if we want to make AI work well on a large scale. Most importantly, using rigorous validation against clinical outcome (rather than comparison to unvalidated clinicians) for safety, efficacy and equity, in a scientifically valid, transparent and accountable way for the whole population, while ensuring patient derived data is used ethically and transparently. Currently there is little training on autonomous AI validation, clinical trials, how to compare to clinical outcome, how to validate human factor design. However, it is essential that as clinicians, all of us become more familiar with understanding whether a specific autonomous AI is the right fit for our patients.
In April 2018, the first-ever autonomous AI was cleared by the FDA. The healthcare system had been entirely built around human physician diagnosing, treating, and recording outcomes, and it was certainly not ready for autonomous AI, where a computer makes a diagnosis or treatment decisions. Regulation, ethics, liability, medical records, payments, and quality measures: none of those aspects were ready to deal with autonomous AI, so we have overcome these hurdles one by one. We created ethical rules for autonomous AI, a pathway for valuing payment for autonomous AI based on the novel concept of “AI work,” how to qualify autonomous AI diagnostic output for clinical quality measures, the legal framework, liability issues, as well as inputting AI into medical records. While we solved most of these, we are still working on some, and they are specific to each country’s healthcare system. There is a lot of enthusiasm for changing systems to incorporate autonomous AI, but there are many aspects to consider – not least safety and efficacy. It is important to be transparent and accountable, and show that you abide by existing rules and regulations.
Keane:It’s important to remember that AI and deep learning are not magic. There are certain applications where deep learning will work very well, but there are many where it won’t. There is no question of AI replacing ophthalmologists.
Certain AI systems might get a better reception in specific healthcare settings. In a public healthcare setting, struggling with huge numbers of patients, it’s going to be much more attractive to have something that prioritizes patients with a sight-threatening disease, so the amount of time spent on people with less serious disease is reduced. In a private healthcare setting, you might not want to reduce the overall number of referrals that are going into the system. Also, any new technology needs a pathway to be introduced into the system.
At Moorfields, we’ve learned how curated data is a major bottleneck for AI projects. So we’ve learned how to aggregate and curate ophthalmic data for the purposes of training AI models, and we’ve introduced the technical infrastructure, so we can actually harness the power of the huge amounts of data that Moorfields produces. We do more than a thousand OCT scans per day at Moorfields, and now we have the infrastructure to deal with them.
We’ve also learned about concerns around using patient data – this is potentially a sensitive topic, which requires caution. Our collaboration involves the use of anonymized clinical data from the NHS in the UK and we are transparent, keeping patients and the public informed about what we’re doing.
We’re trying to create an ecosystem of world-leading AI experts, so that we can do lots of novel, early-stage development of AI systems in an academic setting. We might start a hundred early-stage projects, but only a minority of those will be translated into clinical practice, with an industry collaborator.
Odaibo: The biggest challenge to adoption is the interdisciplinarity of the problem at hand. Communication amongst clinicians, clinical administrators, regulators, data scientists, and data engineers is crucial, but these individuals often speak different languages. The relative novelty of AI technologies also requires some time for everyone to come on board and understand each other’s languages. Regulatory barriers are appropriately in place and pace the deployment into clinical usage. There are also some engineering challenges to overcome to have full spectrum continuity in learning and improvement of systems.
Cost will also play a role; cloud-based systems and AI talent are both costly. All in all, appropriate cross-talk and continued advancement will inevitably result in progress because the overall projected cost-savings and clinical efficacy improvement is significantly compelling.
Ting: The potential challenges of AI research and clinical adoption in ophthalmology are numerous. First, AI approaches in ocular disease require a large number of images. Data sharing from different centers is an obvious approach to increase the number of input data for network training, however, increasing the number of data elements does not necessarily enhance the performance of a network. For example, adding large amounts of data from healthy subjects will most likely not improve the classification of disease. Moreover, very large datasets for training may increase the likelihood of making spurious connections (13). When it comes to using retinal images to predict and classify ocular and systemic disease, a clear guideline for the optimal number of cases for training is needed.
Second, when data are to be shared between different centers, regulations and state privacy rules need to be considered. These may differ between countries, and while they are designed to ensure patients’ privacy, they sometimes form barriers for effective research initiatives and patients’ care. Generally, there is an agreement that images and all other patient-related data need to be anonymized and patient’s consent has to be obtained before sharing, if possible. The implementation of the necessary solutions – including data storage, management, and analysis – is time- and cost-intensive. Investing in data-sharing is a difficult decision, because the financial requirements are high, and the benefit is not immediate. Nonetheless, all AI research groups worldwide should continue to collaborate to rectify this barrier, aiming to harness the power of big data and DL to advance the discovery of scientific knowledge.
Third, the decision for data sharing can sometimes be influenced by the fear that competitors may explore novel results first. This competition can even occur within an institution. Indeed, key performance indicators (as defined by funding bodies or universities, including number of publications, impact factor and citation metrics) may represent major hurdles for effective data sharing. On an institutional level the filing of collaboration agreements with other partners is a long and labor-intensive procedure that slows down analysis of shared data. Such periods may even be prolonged when intellectual property issues are to be negotiated. Given that these are usually multiple-institution agreements, time spans of one year or more are common.
Fourth, a large number of images are required in the training set and they need to be well phenotyped for different diseases. The performance of the network will depend on the number of images, the quality of those images, and how representative the data is for the entire spectrum of the disease. In addition, the applicability in clinical practice will depend on the quality of the phenotyping system and the ability of the human graders to follow that system.
Fifth, though the number of images that are available for diseases such as glaucoma, DR and AMD is sufficient to train networks, orphan diseases represent a problem because of the lack of cases. One approach is to create synthetic fundus images that mimic the disease. This is a difficult task and current approaches have proven unsuccessful (14, 15). In addition, it is doubtful that competent authorities would approve an approach where data do not stem from real patients. Nevertheless, generation of synthetic images is an interesting approach that may have potential for future applications.
Sixth, the capabilities of DL should not be construed as competence. What networks can provide is excellent performance in a well-defined task. Networks are able to classify DR and detect risk factors for AMD, but they are not a substitute for a retina specialist. As such, the inclusion of novel technology into DL systems is difficult, because it will require a large number of data with this novel technology. Inclusion of novel technology into network-based classification systems is a long and costly effort. Given that there are many novel imaging approaches on the horizon, including OCT-angiography or Doppler OCT(16, 17), this may have considerable potential for diagnosis, classification and progression analysis. And that is an important challenge for the future.
Seventh, providing healthcare is logistically complex, and solutions differ significantly between different countries. Implementing AI-based solutions into such workflows is challenging and requires sufficient connectivity. A concerted effort from all stakeholders is required, including regulators, insurances, hospital managers, IT teams, physicians, and patients. Implementation needs to be easy and straightforward, and without administrative hurdles. Quick dissemination of results is an important aspect in this respect. Another step for AI being implemented into a clinical setting is a realistic business model that needs to consider the specific interest of the patient, the payer, and the provider. Main factors to be considered in this respect are reimbursement, efficiency, and unmet clinical need. The business model also needs to consider the long-term implications, because continuous connectivity and the capacity to learn is associated with the ability to improve clinical performance over time.
Eighth, there is a lack of ethical and legal regulations for DL algorithms. These concerns can occur during the data sourcing, product development, and clinical deployment stage (18, 19). Char and colleagues stated that the intent behind the design of DL algorithms also needs to be considered (18). One needs to be careful about building racial biases into the healthcare algorithms, especially when the healthcare delivery already varies by race. Moreover, given the growing importance of quality indicators for public evaluations and reimbursement rates, there may be a tendency to design the DL algorithms that would result in better performance metrics, but not necessarily better clinical care for the patients. Traditionally, a physician could withhold the patients’ information from the medical record to keep it confidential. In the era of digital health records integrated with the deep-learning-based decision support, it would be hard to withhold patients’ clinical data from the electronic system. Hence, the medical ethics surrounding these issues may need to evolve over time.
Lastly, the AI system is supposed to be a cost-effective tool for screening eye diseases. Thus, this may not be the bottleneck when compared with the above-mentioned challenges.
How long before we see real changes in patient outcomes as a result of implementing AI/deep learning?
Abramoff: Autonomous AI solves access, cost and quality problems in places where the diabetic eye exam was previously not accessible for people with diabetes. We have implemented the systems at places where the access time to ophthalmology was half a year or more. After installing autonomous AI, these health systems can now provide patients with same-day eye care appointments. AI has already diagnosed thousands of patients who have been tested for diabetic retinopathy, and a sizeable percentage were found to have diabetic retinopathy, and were therefore referred for further treatment – which we know saves sight. In fact, now that IDx has solved the accessibility and cost problem of the diabetic eye exam, we have been focusing on the full care pathway to ensure improved outcomes.
Things are moving at an incredibly rapid pace. Except for ophthalmology, autonomous AI is not available to the public anywhere: you can’t buy a self-driving car yet, you cannot get a loan from an autonomous unsupervised AI, but you can now get a diagnosis from an AI system. And people are getting diagnosed by AI today. I find it amazing that healthcare was the first field to deploy autonomous AI when we hear so much about autonomous cars.
Keane: I predict it to be a timeframe of two to three years. I think it is coming sooner than we might imagine.
Odaibo: I believe that in about three to five years medicine will be notably different as a result of AI, and the current time will undoubtedly be considered a prior era of medicine.
Ting: We started integrating the AI system into the Singapore Integrated DR Screening Program (SiDRP) in 2018. We are expecting to see the patients’ outcomes in the next three to five years. For other conditions such as glaucoma, AMD or ROP, it may take slightly longer than the DR algorithms, although many groups have already published robust outcomes on the respective AI systems.
What’s exciting in AI/deep learning right now – and which groups or projects are you following around the world?
Keane: I pay more attention to people in the field than to projects, and two people immediately spring to mind. The first is Aaron Lee, an ophthalmologist in Seattle, USA – one of very few doctors in the world with a deep understanding of the technical aspects of AI, combined with a deep understanding of ophthalmology; he’s doing a lot of novel work. The other person is Luke Oakden-Rayner, a radiologist in Australia.
For AI to transform healthcare, professionals have to be central to the process. It cannot be transformed by people outside of the profession, and it is far more powerful to have a healthcare professional who has some knowledge of both worlds, than it is to have a world-leading ophthalmologist with no knowledge of AI or a world-leading AI expert with no knowledge of ophthalmology.
Odaibo: On the data science side, one very exciting area is generative models, such as generative adversarial networks and variational auto-encoders, which are methods that allow one to generate synthetic data, as well as learn the latent features of a representation. On the engineering side, advances in AI DevOps platforms and methods bring us closer to feasible truly-continuous systems. I’m actively involved in this area and RETINA-AI Health will be hosting an AI DevOps hackathon in February 2020 in Lagos in collaboration with Microsoft Africa. I’m also writing a technical tutorial series in Medium on container-orchestration technologies, such as Kubernetes.
Ting: To me, the most exciting advance in AI/deep learning is the evolution of quantum physics published by Arute and colleagues from Google AI (20). The processor that has been described can significantly increase the processing speed for data analysis. It takes about 200 seconds (3.5 minutes) to sample one instance of a quantum circuit a million times – to put this in context, the same task done by a supercomputer at present takes approximately 10,000 years. This technology may well take us to industry 5.0 within the next few decades as it may, once again, disrupt many technical and medical industries.
Does the implementation of AI/deep learning into ophthalmic practice have a competitive element to it?
Odaibo: There absolutely is a competitive element! Country-wise, some of the top contenders in the field of AI in general are the US, China and Russia, while the fastest-growing in terms of talent is in Africa and Nigeria, in particular. In terms of funding and state-level enthusiasm, China is far ahead of every other country. The US is a distant second in funding of AI. Anecdotally, it appears that eight of every ten venture firms looking to invest in AI are based in China. It will be interesting to see how it all plays out.
Ting: It certainly does. US/Canada and Europe have always been running at the forefront of many countries worldwide due to the proximity to all the world class computer science departments (like Cambridge, Imperial, University of Toronto, New York University and the California Institute of Technology). In Singapore, we are also privileged to have a world-class technical team to develop many robust algorithms in ophthalmology. Singapore only has a population of 5 million. It is much smaller than other counties, but also, thanks to this, it is easier to create a robust ecosystem to support the clinical deployment of an AI algorithm – for example, the aforementioned integration of the AI system into the Singapore national DR screening program (2). Nonetheless, if I were to pick which country is going to get there first, China will still be on the top of my list for several reasons. First, China is the country with the largest population worldwide, and in terms of data, they will always beat other countries. Second, people will then question the cleanliness of their data.
In my mind, the top five eye institutions in China are already competing at the world-class level, including AI, and many of the Chinese clinicians are, to me, under-rated. On a recent trip to China, I was shocked to find what they had already achieved in AI and data science in ophthalmology. Third, the Chinese government, led by President Xi, is extremely supportive of making China an AI-integrated society. There are many funding opportunities available for research and development related projects. At present, there are already many ongoing real time AI-integrated algorithms deployed in the clinical space. That being said, while China may still be slightly ahead of the game, the language barrier may hinder some good scientific findings from being accepted in the high-impact medical journals.
What’s next for AI and deep learning in eye care? Do you have a wish list for the next 5-10 years – and beyond?
Abramoff: The biggest problem for bringing new autonomous AI to market right now is agreeing on what the disease actually is. For DR or AMD we have had outcome-based standards for decades: surrogates for outcome. It is easier to rigorously validate an autonomous AI to market when we have such an outcome or surrogate outcome that is widely accepted and evidence based. In glaucoma, we are slowly coming to an appropriate surrogate outcome, and when that is done, we can rigorously validate autonomous AI for glaucoma, and similarly for other diseases.
Keane: Ten years from now, a patient will come in, and we will have 10 different types of high-resolution imaging of the eye, like adaptive optics or OCT, a lot of different functional tests, such as visual field testing, electrophysiological tests, ERGS, as well as full genomic testing. Maybe we have the patient’s metabolomics and their proteomics from a urine sample, and they have uploaded the contents of their phone or smartwatch, so it tells us about their activity and real-world visual function. We will then need AI systems to help us integrate all these complex multi-modal data, so we can make the best decisions for our patients.
I also hope that we can bring the expertise of a hospital like Moorfields into the community and into patients’ homes so that, if they have a stable chronic eye disease like glaucoma, they don’t have to come into the hospital for check-ups. A lot of the monitoring of these chronic diseases could be done in the community and in the homes, improving quality of life. AI will be a big facilitator of that.
Odaibo: Incremental steps are how we will advance. The importance of implementation and engineering will increasingly be appreciated. Additionally, healthcare financing models will develop and mature on a case-by-case basis to gradually inch us forward towards increased healthcare access.
Ting: I would like to see more of the research AI algorithms translated into clinical care over the next few years. Hopefully they will make a positive impact on patient outcomes and experience. The application of deep learning in genomics and proteomics is also something I am eagerly anticipating. Google Deep Mind Alphafold team has won the Critical Assessment of protein Structure Prediction (CASP) in 2018 and, since then, multiple research groups have explored the use of deep learning in similar domains. The implications of selecting the appropriate target gene or proteins may bring many breakthroughs in novel therapeutic targets, and could potentially halt or even reverse some incurable diseases.

- MD Abràmoff et al., “Pivotal trial of an autonomous AI-based diagnostic system for detection of diabetic retinopathy in primary care offices”, NPJ Digit Med, 1 (2018). PMID: 31304320.
- D Ting et al., “Development and validation of a deep learning system for diabetic retinopathy and related eye diseases using retinal images from multiethnic populations with diabetes”, JAMA, 318, 2211 (2017). PMID: 29234807.
- D Ting et al., “AI for medical imaging goes deep”, Nat Med, 24, 539 (2018). PMID: 29736024.
- D Ting et al., “Deep learning in estimating prevalence and systemic risk factors for diabetic retinopathy: a multi-ethnic study”, NPJ Digit Med, 2, 24 (2019). PMID: 31304371.
- D Ting and T Wong, “Eyeing cardiovascular risk factors”, Nat Biomed Eng, 2, 140 (2018). PMID: 31015720.
- V Bellemo et al., “Artificial intelligence using deep learning to screen for referable and vision-threatening diabetic retinopathy in Africa: a validation study”, Lancet Digital Health, 1, 35 (2019).
- D Ting et al., “Deep learning in ophthalmology: The technical and clinical considerations”, Prog Retin Eye Res, 72, 100759 (2019). PMID: 31048019.
- D Ting et al., “Artificial intelligence and deep learning in ophthalmology”, Br J Ophthalmol, 103, 167 (2019). PMID: 30361278.
- SG Finlayson et al., “Adversarial attacks on medical machine learning”, Science, 363, 1287 (2019). PMID: 30898923.
- Y LeCun et al., “Deep learning”, Nature, 521, 436 (2019).
- D Ting et al., “An Ophthalmologist’s Guide to Deciphering Studies in Artificial Intelligence”, Ophthalmology, 126, 1475 (2019). PMID: 31635697.
- JQ Li et al., "Prevalence and incidence of age-related macular degeneration in Europe: a systematic review and meta-analysis", Br J Ophthalmol (2019). PMID: 31712255.
- L Gomes, “Machine-learning maestro Michael Jordan on the delusions of big data and other huge engineering efforts”, IEEE Spectrum, 20 (2014).
- E Menti et al., “Automatic Generation of Synthetic Retinal Fundus Images: Vascular Network”, Cham: Springer International Publishing, 167 (2016).
- S Fiorini et al., “Automatic Generation of Synthetic Retinal Fundus Images: Vascular Network”, Procedia Computer Science, 90, 54 (2016).
- V Doblhoff-Dier et al., “Measurement of the total retinal blood flow using dual beam Fourier-domain Doppler optical coherence tomography with orthogonal detection planes”, Biomedical optics express, 5, 630 (2014). PMID: 24575355.
- R Leitgeb et al., “Doppler optical coherence tomography”, Progress in Retinal and Eye Research, 41, 26 (2014).
- D Char et al., “Implementing Machine Learning in Health Care - Addressing Ethical Challenges”, The New England journal of medicine, 378, 981 (2018).
- E Vayena et al., “Machine learning in medicine: Addressing ethical challenges”, PLoS Med, 15, e1002689 (2018). PMID: 30399149.
- F Arute et al., “Quantum supremacy using a programmable superconducting processor”, Nature, 574, 505 (2019). PMID: 31645734.
- FDA, "Proposed regulatory framework for modifications to artificial intelligence/machine learning (AI/ML)-based software as a medical device (SaMD)" (2019). Available at: bit.ly/2Lfs40D. Accessed December 4, 2019.
- WHO, "WHO Guideline: recommendations on digital interventions for health system strenghtening" (2019). Available at: bit.ly/2rP1W63. Accessed December 4, 2019.
A specialist in in applied clinical imaging research, Pearse has a particular interest in optical coherence tomography. Now a consultant at Moorfields Eye Hospital, he previously carried out OCT research with some of the original inventors of the technology at the Doheny Eye Institute. As the first UK ophthalmologist to receive a Clinician Scientist Award from the National Institute of Health Research, his current work focuses on exploring the potential of new technologies and innovations in the treatment of visual impairment and blindness.
Stephen Odaibo is a retina specialist, computer scientist, full-stack AI engineer and co-founder of RETINA-AI.
Vitreoretinal Specialist at the Singapore National Eye Center, Assistant Professor of Ophthalmology at Duke-NUS Medical School, Singapore, and Adjunct Professor at the State Key Laboratory of Ophthalmology at Zhongshan Ophthalmic Center in China.