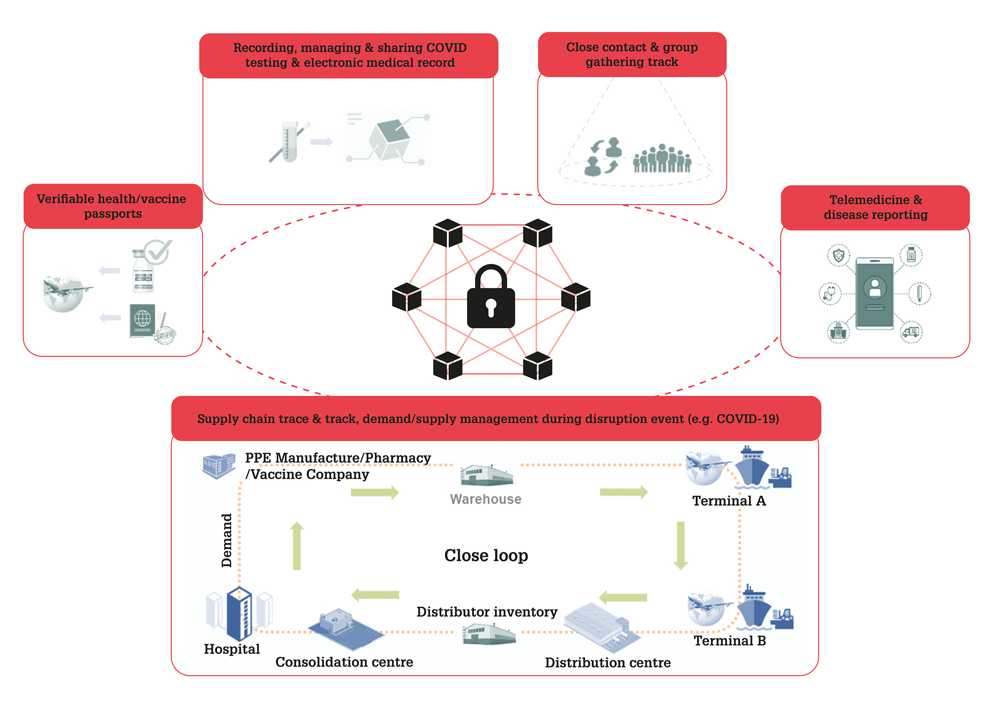
Artificial intelligence (AI) is one of the key digital technologies that are expected to shape our future healthcare model (1, 2). The ophthalmic fraternity is experiencing rising optimism over the viability of AI being integrated into clinical ophthalmic care following recent successes in AI diabetic retinal photography assessment. The availability of Big Data allows AI models to be effectively trained to perform a variety of roles such as clinical decision support, virtual nursing assistance and automated diagnosis. Naturally, data as well as model architecture remain the key ingredients for the development of a robust AI algorithm. Conversely, these also present challenges that could create a bottleneck, limiting the viability of such systems. These include restrictions arising from data governance as well as trust and explainability concerns pertaining to the black-box architecture. Obstacles to data transparency and transferability not only limit multi-center collaboration but also negatively impacts user confidence.
Blockchain on the other hand is a form of distributed ledger (transaction recording) technology that emphasizes decentralization, transparency, immutability and auditability. Each transaction is recorded in a block which is linked cryptographically to the previous block via the preceding block’s hash key. Metadata, such as data provenance, contributes to the derivation of the hash key and is similarly stored. This unique identifier is analogous to the fingerprint as it is both collision-resistant and deterministic. Each block undergoes a consensus process by participating stakeholders, known as nodes, before it can be verified, approved and stored on the chain. This costless verification results in an ever-growing chain of readable but immutable transactions. Popular consensus mechanisms found in most mainstream blockchain platforms include Proof of Work (Bitcoin), Proof of Stakes (Ethereum) and Practical Byzantine Fault Tolerance (Hyperledger). Blockchain platforms are also differentiated based on participation and access-rights. These include public (permissionless), private (permissioned with a single authority) and consortium blockchains (permissioned with multiple governing organizations), of which the latter two are better suited for healthcare implementation.
Blockchain in healthcare
The meteoric rise of cryptocurrency globally has generated significant interest in the utility of blockchain. As acceptance increases, the utility of blockchain has expanded beyond the usual financial remits. The healthcare sector could be poised for disruption if blockchain technology achieves greater traction. This potential was not only aptly highlighted in the 2019 technical report by the International Telecommunication Union – Telecommunication Standardization Sector (3), but has also gained new impetus with the onset of the COVID-19 pandemic. One of the most widely explored indications involves the management and access control of electronic health records. A new paradigm of patient-centric care is achieved through decentralization and cryptography, enabling peer-to-peer transaction via patient-controlled access. This creates a new mode of medical data flow that is both traceable and secure, allowing true democratization of healthcare data. In comparison, traditional healthcare databases with client-server architectures are dependent on a central approving authority. This not only greatly limits efficient data exchange, but also creates a single point of failure that could be exploited by malicious actors.
The challenges brought about by the COVID-19 pandemic have also emphasized the need for verifiable data exchange. The case for blockchain-enabled pandemic management is clearly demonstrated by two unique scenarios – immunity or health certificates (4), and vaccine supply chain management (5). Secure cross-border exchange of data that is immutable, auditable and verifiable is critical to the success of pandemic control. Fraudulent activities such as counterfeit vaccines and forged immunity certificates are deterred in view of the transparency and traceability afforded by blockchain technology. This in return ensures proper movement control monitoring, robust contact tracing and transparent vaccine management.
Decentralized solution
Ubiquitous measures adopted to control the pandemic, such as social distancing and movement control, have also encouraged decentralization of healthcare services. These have primarily employed remote monitoring, teleconsultation as well as the integration of Medical Internet of Things (MIoT) (6). These innovative models of care not only reduced the strain on hospitals by distributing healthcare services into the community, but also deliver a measure of convenience and scalability that was lacking previously. Similarly, clinical research protocols that were previously institution-centric have been compelled to adopt remote monitoring in a bid to negotiate pandemic-induced disruptions. To achieve these, it is necessary to ensure efficient transfer and data integrity between stakeholders. In this sense, blockchain provides a ready decentralized solution by leveraging cryptography and encryption techniques to support peer-to-peer networking and costless verification. This allows medical data to be transacted in a secure purposeful manner both offline and online in spite of the need for physical separation.
The impetus for decentralization experienced in the financial sector can transform the healthcare insurance sector as well (7). In the current state, there is a lack of visibility of a patient’s coverage amongst healthcare insurance providers, leading to overlapping coverage that could lead to duplicative claims. Compounding this are fraudulent claims for frivolous or excessive treatments by either patient or healthcare provider which are difficult to detect, resulting in significant losses for insurance providers. Furthermore, there is a lack of a direct communication channel between the hospital, patient and insurance provider. Coordinating between multiple parties often proves to be a long-drawn process, resulting in significant delay before a claim can be approved. Blockchain on the other hand has the potential to bridge this gap. Through peer-to-peer networking, confidential healthcare data and insurance policies can be accessed directly by pre-approved nodes, providing clarity to participating healthcare insurance vendors simultaneously. Separately, the adjudication process for each medical claim could also be expedited using blockchain smart contracts. Typically, each claim undergoes a time-consuming and laborious process involving several rounds of review prior to approval. Removing these steps through smart contract automation would significantly improve the efficiency and turnaround time for the insurance provider, reducing needless anxiety for the policyholders.
Artificial intelligence and blockchain
Recently, we conducted a systematic review that was published in Lancet Digital Health, focusing on the research and development of blockchain health care applications (8). After examining studies published across six major scientific databases, one of the main areas of application identified was AI development and big data management. Recent advancements made in AI have highlighted the importance of the accessibility to big data. Without a critical mass of data, it is difficult to develop robust AI models that can be translated clinically. Many of these models often achieve good results during internal validation, but often suffer from poor generalizability and performance when exposed to the real world. The main constraint afflicting researchers globally is the lack of access to the required data, due to the fact that these are often highly confidential. The enactment of stringent data privacy laws such as the Health Insurance Portability and Accountability Act as well as General Data Protection Regulation creates an access barrier that is sometimes difficult to surmount. Furthermore, the stringent and often complicated bureaucracy prevents proper data sharing between various research sites, effectively prohibiting cross-institution or cross-border collaboration. One of the solutions to this problem is to create open-source data. However, the drawbacks include variable dataset quality, dataset scarcity, lack of variation of disease types especially for rare or orphan diseases and need for maintenance. Furthermore, overuse of these sources could lead to convergence in performance of the AI models that might not be translatable and generalizable.
In order to avoid the need for data transfer, an alternative privacy-preserving approach would be required. Federated learning (FL) combined with a blockchain infrastructure has been proposed as a likely solution. This technique involves decentralized training by various nodes using their respective local dataset, obviating the need for dataset transfer. During the training, the parameters of each local model are then updated to a central server. As private information is still contained within the gradients shared with the central server, FL in itself only guarantees confidentiality. Additional privacy-dedicated algorithms, such as Differentially-Private Stochastic Gradient Descent, will need to be incorporated before full privacy protection is achieved. Blockchain as a platform plays a key supporting role by managing trust, recording every detail of the development immutably (including algorithms and parameters), authenticating each participating node, coordinating the training using smart contracts and managing the reputation of the nodes (9). Provenance of the data is also recorded immutably on the blockchain for verification purposes, something which FL alone is unable to provide. In addition, the blockchain can enable real-time processing of medical data while concurrently orchestrating different tasks amongst the nodes to minimize operation time. This is particularly promising for FL in multi-agent systems that involve MIoT (10). More recently, Warnat-Herresthal and colleagues went beyond FL and proposed a swarm learning model supported by blockchain peer-to-peer networking to achieve complete decentralization of algorithm training (11). The blockchain served several functions, including enrolling new nodes using smart contracts, peer-to-peer exchange of model parameters via application programing interface while providing robust measures against malicious nodes.
Aside to data privacy, another major challenge faced during the development of AI models is the visibility of the entire process. This includes stepwise weight and parameter refinement, interim results and datasets management. It is common practice to report the final results while the granular details throughout the developmental process remains in a black box. Such practices unfortunately can have a negative impact on trust and explainability of the AI algorithms. In a recent study by Tan and colleagues, the group comprehensively illustrated the role of blockchain in promoting secure and transparent AI development under real world conditions (12). Using images of myopic individuals, a deep learning system was first developed to detect the presence of myopic maculopathy. A blockchain platform designed on the Hyperledger Fabric 2.0 architecture was next implemented to first coordinate secure image handling across three separate sites in two countries, followed by model sharing with validation results and finally on-chain recording of external testing performance. Independent parties that wish to assess the veracity of the algorithm development can verify based on the immutable hash values. This could provide healthcare regulatory bodies (such as US Food and Drug Administration or European CE regulatory body) with a more efficient and effective model to evaluate clinical AI algorithms.
Conclusion
As acceptance of digital technology gains pace, both AI and blockchain could begin to play a significant role in shaping the future of global healthcare. However, the development of healthcare AI still face key bottlenecks related to data, privacy and trust. In order to overcome these key challenges, the community should leverage on the unique advantages provided by blockchain, such as decentralization, security, and auditability. This digital infrastructure could possibly be the key element that will support greater collaborative research, improve transparency and enhance privacy protection.
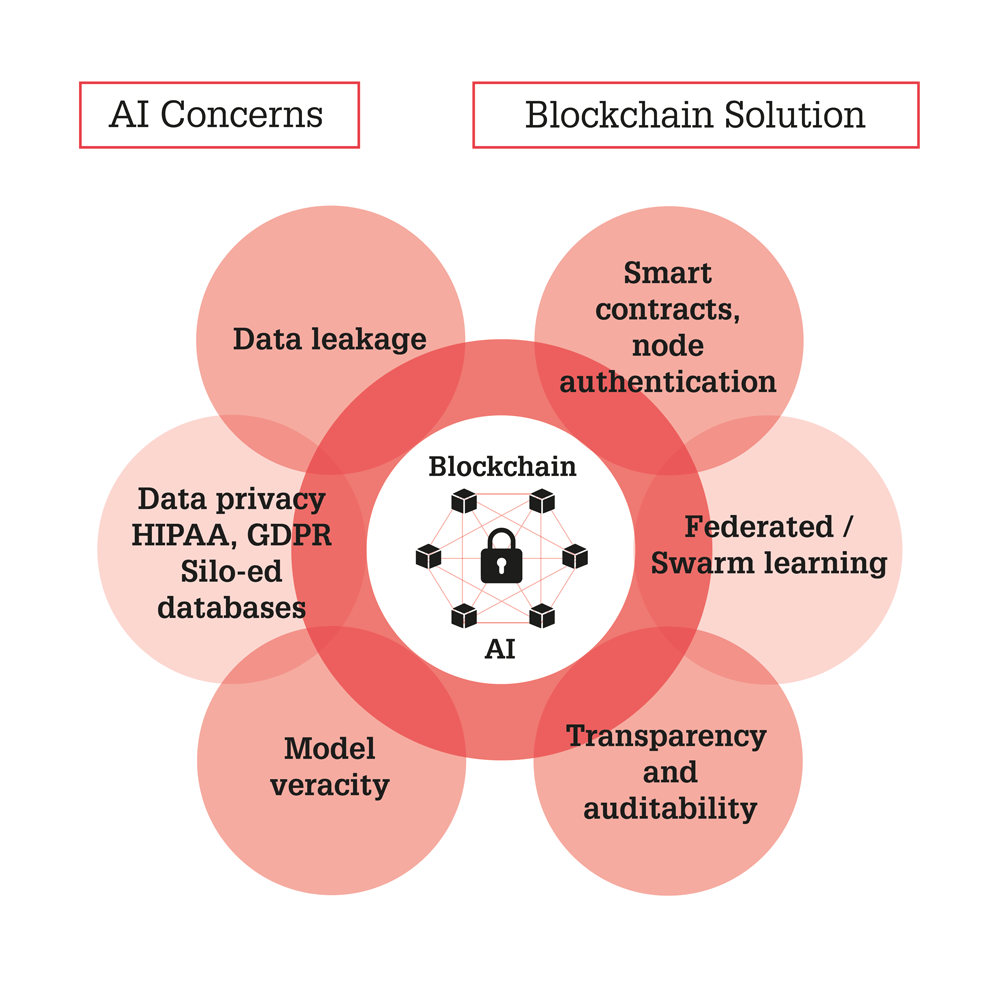
References
DSW Ting et al., "Artificial intelligence, the internet of things, and virtual clinics: ophthalmology at the digital translation forefront," The Lancet Digital Health, 2, e8 (2020).
-
JO Li et al., "Digital technology, tele-medicine and artificial intelligence in ophthalmology: A global perspective," Progress in retinal and eye research, 82 (2021).
-
International Telecommunication Union Telecommunication Standardization Sector, "Distributed ledger technology use cases" (2019).
-
A Abid et al., "NovidChain: Blockchain-based privacy-preserving platform for COVID-19 test/vaccine certificates," Software: practice & experience (2021).
-
RW Ahmad et al., "Blockchain-Based Forward Supply Chain and Waste Management for COVID-19 Medical Equipment and Supplies," IEEE Access, 9, 44905 (2021).
-
AD Dwivedi et al., "A Decentralized Privacy-Preserving Healthcare Blockchain for IoT," Sensors, 19 (2019).
-
TK Mackey et al., "Combating Health Care Fraud and Abuse: Conceptualization and Prototyping Study of a Blockchain Antifraud Framework," Journal of medical Internet research, 22, e18623 (2020).
-
WY Ng et al., "Blockchain applications in health care for COVID-19 and beyond: a systematic review," The Lancet Digital Health (2021).
-
MA Rahman et al., "Secure and Provenance Enhanced Internet of Health Things Framework: A Blockchain Managed Federated Learning Approach," IEEE Access, 8, 205071 (2020).
-
D Połap et al., "Agent architecture of an intelligent medical system based on federated learning and blockchain technology," Journal of Information Security and Applications, 58 (2021).
-
S Warnat-Herresthal et al., "Swarm Learning for decentralized and confidential clinical machine learning," Nature, 594, 265 (2021).
-
TE Tan et al., "Retinal photograph-based deep learning algorithms for myopia and a blockchain platform to facilitate artificial intelligence medical research: a retrospective multicohort study," The Lancet Digital Health, 3, e317 (2021).