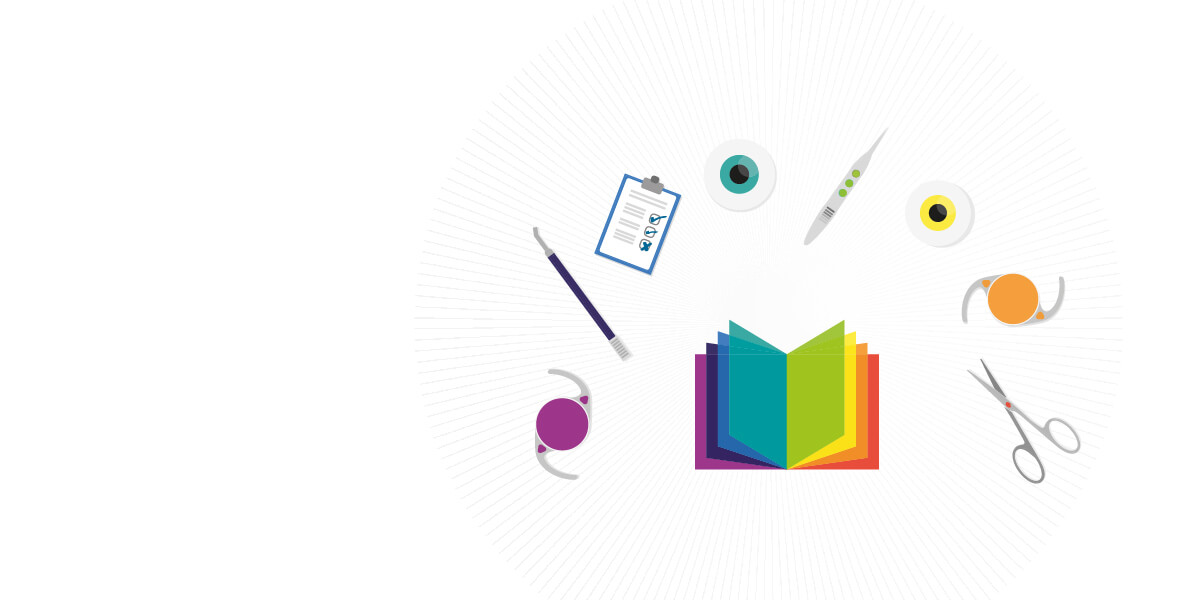
Landmark paper: JM Brown et al., “Automated Diagnosis of Plus Disease in Retinopathy of Prematurity Using Deep Convolutional Neural Networks”, JAMA Ophthalmol, 136, 803-810 (2018). PMID: 29801159.
Retinopathy of prematurity (ROP), a retinal vasoproliferative disease affecting premature infants, is a leading cause of childhood blindness worldwide. Standard clinical criteria have been established for diagnosis and treatment, and severe ROP can be successfully treated – if it is diagnosed early. The Early Treatment for Retinopathy of Prematurity multicenter clinical trial showed that “plus disease” is the most important parameter for identifying severe treatment-requiring ROP. Plus disease is defined as arterial tortuosity and venous dilation in the posterior pole, and accurate and consistent diagnosis of plus disease is critical to ensure that infants at risk of blindness receive the appropriate treatment. An intermediate stage – the pre-plus category – is defined as retinal vascular abnormalities that are insufficient for plus disease, but have more arterial tortuosity and venous dilation than normal.
Traditionally, ROP screening has been carried out in neonatal intensive care units (NICUs) using indirect ophthalmoscopy, or by obtaining retinal images using a contact fundus camera, with grading by a pediatric ophthalmologist or retinal specialist. The process is very time consuming, and the quality is variable. Recently, artificial intelligence (AI) including deep learning technology has been applied to fundus photographs and OCT images for accurate diagnosis of common adult retinal diseases (1, 2, 3). Within this context, it is natural to consider applying a similar approach to ROP screening to identify plus disease – Brown and colleagues did exactly that in my choice of “landmark literature.” Despite a relatively small sample size in training and testing, outcome of a deep classification model performed very well, as demonstrated by the ROC curves generated for detecting plus disease and pre-plus disease. It is apparent that deep learning is well suited to this task. A common problem that plagues most deep learning studies is the lack of an accurate and pristinely-labeled dataset; however, this study did not suffer from such a problem, as the diagnosis and image quality were independently reviewed by three trained graders, and, more importantly, determined by an experienced ophthalmologist after a full evaluation in the NICU. As the study involved a sequential pass through a fully-convolutional U-Net model before the classification model, the diagnosis was not made upon the original image itself, but rather a black-and-white “mask” where the blood vessels were colored white, and the rest of the image was colored black, ignoring all information other than the shape of the blood vessels. It is very exciting to see that all the information the deep learning model in this paper used to make an accurate diagnosis was found in the width, orientation, and tortuosity of the vessels. However, as the dataset was rather small (approximately 5500 eye exams), it is difficult to determine if this method can reliably mimic physician performance in a variety of real-world scenarios. On the other hand, given that there is a wide variation in quality and consistency when grading the same ROP photographs among very experienced physicians, the method is expected to out-perform physicians when given a large dataset (and trained and validated in a variety of clinical settings). I believe this method has the potential to be a great tool in aiding ROP clinical care.
References
- 1. V Gulshan et al.,“Development and Validation of a Deep Learning Algorithm for Detection of Diabetic Retinopathy in Retinal Fundus Photographs”, JAMA, 316, 2402-2410 (2016). PMID: 27898976. 2. DSW Ting et al., “Development and Validation of a Deep Learning System for Diabetic Retinopathy and Related Eye Diseases Using Retinal Images from Multiethnic Populations with Diabetes”, JAMA, 318, 2211-2223 (2017). PMID: 29234807. 3. DS Kermany et al., “Identifying Medical Diagnoses and Treatable Diseases by Image-Based Deep Learning”, Cell, 172, 1122-1131 (2018). PMID: 29474911.