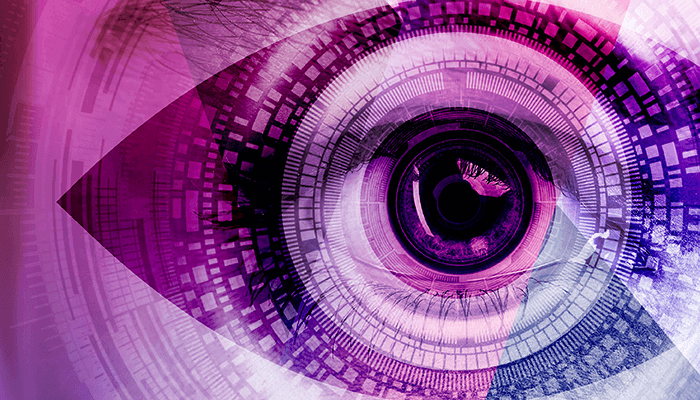
Technological advances in artificial intelligence (AI) have progressed significantly in recent years across many different fields. Ophthalmology is no exception and there has been increased interest in the use of deep learning (DL) models to screen and interpret clinical findings. Given that there are many rural locations with few doctors across the globe, AI tools can not only ensure lower costs but also remove barriers that limit access to care for far too many people (1).
It has been shown that DL can be adapted for the detection of retinal lesions, including diabetic retinopathy (2). Diabetes is a growing epidemic; approximately 400 million adults had been diagnosed by 2017, and this is projected to reach over 600 million by 2044. Of these patients, approximately one-third will develop some form of retinopathy – and 10 percent are likely to develop sight-threatening retinopathy (1). These are worrying numbers and numerous studies have already looked into using DL to detect and classify diabetic retinopathy from retinal photos to anticipate diagnosis and provide early intervention. Many other studies have also looked at other retinal vascular diseases (3, 4). However, diseases in the peripheral region are often cut off in standard fundus photos and, considering that up to 40 percent of eyes have diabetic retinopathy occurring in the periphery, there is a clear need to use ultra-widefield (UWF) imaging to capture as much information about the retina as possible (5). Furthermore, in eyes without any preexisting proliferative diabetic retinopathy (PDR), the presence of peripheral retinopathy signifies an almost fivefold increased risk for progression to PDR (6). Many other retinal conditions overlap with the appearance of DR and can make diagnosis tricky; namely, retinal vein occlusions (RVO) and sickle cell retinopathy (SCR).
At the Centre Hospitalier Intercommunal de Créteil (CHIC, France), Dr Alexandra Miere and our team specializing in retina was one of the first to test whether DL can learn to distinguish among different types of retinal vascular diseases using UWF color fundus photography (UWF-CFP). Moreover, UWF-CFP mitigates the disadvantages of having small standard fundus photos, allowing us to capture more of the pathology which, in turn, increases our analytical accuracy.
Applying DL program to retrospective UWF-CFP images
In our study, we performed a retrospective analysis of 224 patients that had UWF-CFP images taken over the last few years (7). The use of UWF allows a 200° field of view when capturing the retina, versus the 30-50° field of a standard fundus photo, so we needed to ensure that peripheral findings were incorporated into our analysis to provide greater information regarding the severity of diseases. In the literature, UWF imaging has been shown to reveal almost 4x more non-perfusion, almost 3x more neovascularization, and almost 4x more pan-retinal photocoagulation compared with standard fundus imaging (6). Before initiating the programming of the DL framework, we grouped the photos into four categories: DR (65), SCR (57), RVO (47), and healthy controls (55). We used the TensorFlow DL framework and took precautions to ensure accuracy throughout the programming: close monitoring of the learning rate, inputting manual adjustments as needed, repeating training, and validity testing. Overall, we found that the classification accuracy was approximately 88 percent, with some groups performing better than others. Only 3 of 57 SCR images and 10 of 47 RVO images were misclassified; however, 21 of 65 DR and 18 of 55 controls were mistakenly classified. The accuracies in SCR and RVO are promising, and highlight the potential of DL to diagnose these cases. Though its application to cases of DR and healthy controls requires further studies, we believe our work underlines the promise of using DL with UWF imaging for diagnosing and classifying retinal conditions.
The future of UWF imaging coupled with AI: prospects and limitations
With a rise in DR cases, retinal photo telehealth services will address a huge global need; in this regard, being able to distinguish DR from other retinal vascular conditions will be pivotal. However, one disadvantage of telehealth is the possibility of missed lesions or erroneous reading caused by limited fields or poor quality images. This problem worsens when trying to implement a DL program as most do not have the capability to troubleshoot beyond their programmed capacity. Before attempting to apply any DL algorithm to classify and interpret retinal images, the images should be of sufficient quality and reveal a major portion of the fundus. In our case, we were able to take care of those concerns using UWF imaging, increasing the visibility of the pathology as well as the accuracy of our DL training. Some other limitations we faced included capturing artifacts (for example, eyelids or lashes) and minor optical limitations when using red-green scanning lasers. Given that this was a retrospective study, there was no way to remove or prevent such artifacts from appearing in the images. However, if we were to repeat our study, it would be very easy to have the participants open their eyes wider or manipulate their ocular structure to remove any artifacts. Multiple images should also be taken to fully take advantage of the quick (<1 second) capture feature. If we continue to increase the responsibilities that AI and DL programs have in terms of handling our ocular health, we need to ensure the best and most complete data set available for analysis.
The future seems bright for AI applied to retinal imaging and diagnosis. The sample cohort of 224 images, though small, allowed for initial results to establish the performance of the AI at this early stage. The integration of cloud storage with artificial intelligence will multiply the images available exponentially, thus allowing the software to learn and refine its ability to detect conditions with precision. Human interpretation will not be replaced, but the first steps of the more digitized process are now available – especially across locations where the number of available ophthalmologists or retina specialists are limited.
References
- A. Grzybowski et al., “Artificial intelligence for diabetic retinopathy screening: a review”, Eye, 34, 451 (2020). PMID: 31488886.
- S. Sengupta et al., “Ophthalmic diagnosis using deep learning with fundus images - A critical review”, Artificial Intelligence in Medicine, 102, PMID: 31980096.
- D. Nagasato et al., “Deep-learning classifier wide ultrawide-field fundus ophthalmoscopy for detecting branch retinal vein occlusion", Int J Ophthalmol, 12, 94 (2019). PMID: 30662847.
- S. Cai et al., “Deep learning detection of sea FAN neovascularization from ultra-widefield color fundus photographs of patients with sickle cell hemoglobinopathy,” JAMA Ophthalmol, 139, 206 (2021). PMID: 33377944.
- M.M. Wessel et al., “Ultra-wide-field angiography improves the detection and classification of diabetic retinopathy”, Retina, 32, 785 (2012). PMID: 22080911.
- P.S. Silva et al., “Peripheral lesions identified on ultrawide field imaging predict increased risk of diabetic retinopathy progression over 4 years”, Ophthalmology, 122, 949 (2015). PMID: 25704318.
- E. Abitbol et al., “Deep learning-based classification of retinal vascular diseases using ultra-widefield color fundus photographs,” BMJ Open Ophthalmol, 7 (2022). PMID: 35141420.