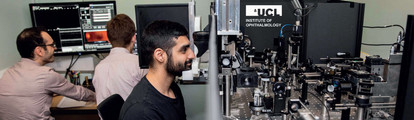
Thoroughly Modern Medicine
Retinal imaging in the era of personalized medicine
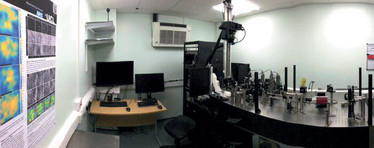
The Adaptive Optics Scanning Laser Ophthalmoscope (AOSLO) is used to image individual cells at the back of the living human eye, namely cone and rod photoreceptors. Such cells are about 50 times smaller than the width of a human hair.
We are lucky to have unprecedented multimodal abilities to non-invasively image the retina these days. Understandably, this has transformed the practice of many retinal physicians and vision scientists. And yet, though our capabilities may be the envy of most other subspecialties, personalized medicine introduces a number of challenges for applications of retinal imaging in ophthalmology.
A major issue is that imaging is still not routine in unaffected or “normal” individuals. This knowledge gap is problematic; to understand whether a feature in a given image represents pathology or just a normal variant demands the availability of patient-specific normative databases. By this we mean that the demographics of the reference or normative database needs to match that of the patient – one cannot necessarily compare image features from a 68-year-old black man to normative data comprised of white women aged 19-23 years of age. The field remains surprisingly naïve with respect to the need for stratifying normative data by age, gender and race. There may well be certain features in retinal images that clearly signify active disease (such as intraretinal fluid viewed on optical coherence tomography), but the utility of images to detect subtle and early changes associated with pathology is limited by the quality of the image and the robustness of the reference database.
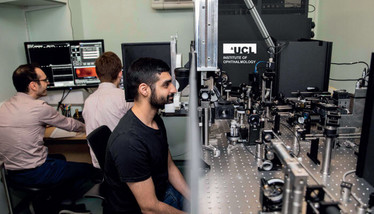
A second important challenge is that the information contained within images is currently extracted in either a qualitative fashion (by “expert” grading) or quantitatively through the use of segmentation or analysis algorithms. Why is that problematic? Because imaging technology continues to evolve exponentially, meaning that clinicians and researchers are constantly re-inventing the wheel when it comes to analysis and interpretation of images. What works for one imaging modality won’t necessarily work for another one (compare segmenting lesions on color fundus images versus segmenting lesions on en face OCT images). Even within a single modality, analysis and interpretation of images can be variable. Logically, an OCT image of the same retina from six different commercial devices contains the same information – but whether a grader or algorithm extracts the same information is variable. What happens when patients switch providers or when a clinic changes devices? We risk potentially compromising the ability to make confident longitudinal assessments on a given patient.
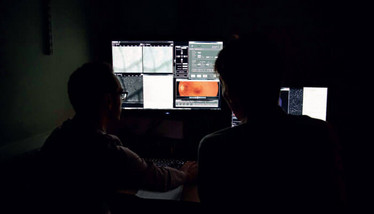
In addition, related to longitudinal imaging, there is a lack of prospective protocol-driven natural history data for the vast majority of retinal conditions. Such datasets are invaluable in the development of image-based biomarkers that could be used to predict progression or even detect the disease. Besides applying natural history studies to more conditions, such studies should be comprehensive in the imaging modalities used. Moreover, most of these studies only deal with monitoring progression after diagnosis. It is exceptionally rare to have data that exists prior to and after diagnosis. However, this is exactly the type of data that could fuel powerful AI approaches.
AI is being increasingly applied to a growing number of retinal imaging studies, seeking to identify features within an image or to classify images by disease type. But the holy grail of personalized medicine is the ability to identify, for example, whether changes in a given individual represent normal aging changes or whether they are likely to progress to age-related macular degeneration. Though there are environmental and genetic “risk factors,” ultimately, it stands to reason that the answer for an individual patient rests within their retinal imaging. The challenge for researchers and AI algorithms is to extract this information in a reliable, sensitive and robust way.
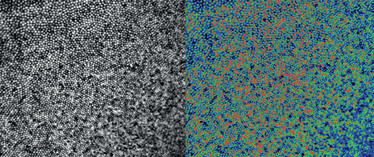
Cone photoreceptors from a young adult unaffected eye.
Moorfields experts share their vision
Michel Michaelides is Professor of Ophthalmology and Consultant Ophthalmic Surgeon, UCL Institute of Ophthalmology and Moorfields Eye Hospital