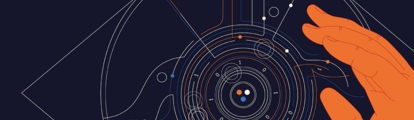
The Algorithms of Power: Part Two
Gurus of ophthalmic AI – Linda Zangwill, Michael F. Chiang, and Damien Gatinel – consider where and how the technology can help deliver the highest quality eye care
Andrzej Grzybowski, Aleksandra Jones | | 9 min read | Interview
To read Part One of this feature, click here

Andrzej Grzybowski, Professor of Ophthalmology and Chair of Department of Ophthalmology, University of Warmia and Mazury, Olsztyn, Poland, and Head of Institute for Research in Ophthalmology, Foundation for Ophthalmology Development, Poznań, Poland
Our virtual AI in Ophthalmology Meeting in June 2022, sponsored by the Polish Ministry of Science and Education, turned out to be a great success, with over 600 registrations from over 20 countries, and lectures delivered by world-leading specialists in this field. I have received many requests to repeat the event next year.
As collaboration and networking of people interested in future applications of AI in ophthalmology is vitally important, I decided to start building the foundations for the International AI in Ophthalmology Society (IAIOph).
Everyone is welcome to join it directly at iaisoc.com or by emailing me: [email protected].
All the lectures from the 2022 AI in Ophthalmology Meeting are available at aiinophthalmology.com.
AI and Glaucoma
Linda Zangwill, Professor of Ophthalmology in Residence, Richard K. Lansche and Tatiana A. Lansche Endowed Chair, Co-Director of Clinical Research, Hamilton Glaucoma Center Director, Data Coordinating Center, Shiley Eye Institute, UC San Diego, California, USA
Why should AI be used in glaucoma? What are the benefits of AI in this field?
There are numerous benefits to using AI to assist in clinical decision making for glaucoma detection and management. AI can improve the accuracy and consistency of glaucoma detection across all levels of ophthalmic care. It can also be used to detect individuals with progressive glaucoma who are in need of closer follow-up and conversely suggest that a patient’s glaucoma is stable and requires less frequent follow-up. In addition, by providing information on the probability of glaucoma, the clinician can integrate this information into their patient management decisions. Moreover, AI can help to screen high-risk individuals for glaucoma in primary care and community settings, so that the disease can be diagnosed in its earliest treatable stages.
Do we know which AI algorithms are better: those based on OCT or those developed on fundus images?
AI algorithms for glaucoma detection based on OCT and fundus images both have high diagnostic accuracy (1,2). Both are useful as they can be valuable in different settings. OCT is the standard of care for the clinical management of glaucoma in most ophthalmology clinics. However, in many communities, particularly in underserved areas, fundus photography is much more likely to be available than OCT imaging. Moreover, glaucoma detection using fundus photography can be integrated with screening for other eye diseases such as diabetic retinopathy and macular degeneration more easily in primary care settings. It is therefore important to develop accurate AI algorithms for both OCT and fundus photography.
Might AI-based glaucoma detection algorithms be used in glaucoma screening in the future?
I believe that AI-based glaucoma detection algorithms will be used for targeted glaucoma screening of high-risk individuals in primary care and/or community settings. Glaucoma screening will likely be integrated with existing algorithms for detection of diabetic retinopathy and other eye diseases. As AI algorithms provide a probability of glaucoma, the cut-off used to refer for follow-up ophthalmic examination can be set to a high specificity needed for screening tests. It should be noted that the US Preventive Services Task Force on Screening for Primary Open Angle Glaucoma recently concluded in the general population of asymptomatic adults 40 years and older “the current evidence is insufficient to assess the balance of benefits and harms of screening for primary open-angle glaucoma” (3). For these reasons, integration of the glaucoma screening with other eye diseases, and targeted screening to high-risk populations should be considered.
Retinopathy of Prematurity Perspective
Michael F. Chiang, Director, National Eye Institute, National Institutes of Health, Bethesda, Maryland, USA
Why was there a need for a new retinopathy of prematurity (ROP) classification?
Interestingly, the early studies on ROP, then called retrolental fibroplasia, were conducted by Thaddeus S. Szewczyk, an American ophthalmologist of Polish origin, who is mostly forgotten today. He was the first to indicate a relationship between the development of ROP and high exposure to oxygen in an incubator or by withdrawing oxygen too rapidly. What has changed in understanding of the ROP pathophysiology in recent 50 years?
We’ve recently published the third version of the international ROP classification system because a number of new challenges since the previous in 2005 have arisen: (i) concerns about subjectivity in critical elements of ROP disease classification such as plus disease and zone, (ii) innovations in ophthalmic imaging and artificial intelligence, (iii) novel pharmacologic therapies (such as anti-VEGF agents) with unique regression and reactivation features post-treatment compared with laser photocoagulation, and (iv) recognition that patterns of ROP in some regions of the world did not neatly fit into the previous classification system.
Why is ROP such a promising area for AI-based medical devices?
First, there are unmet needs in ROP care worldwide, such as workforce challenges, subjectivity of clinical diagnosis, and significant medical legal liability. Second, there is an existing international ROP classification system so there is a standard method of clinical diagnosis that is used worldwide. Third, there is a standard approach to clinical management based on decades of multicenter collaborative clinical trials such as CRYO-ROP, ETROP, and BEAT-ROP. Finally, there are pediatric retinal imaging devices coupled with infrastructure to capture clinical diagnosis and outcome data. Taken together, these factors create a good environment for artificial intelligence implementation and evaluation research studies.
What do we know about problems related to differences between graders?
Classically, we diagnose ophthalmic diseases by examining morphology of the eye. These clinical observations are typically qualitative, and we often convert those morphological observations into structured classifications (for example, “stage 1” or “plus disease” in ROP, “neovascularization elsewhere” in diabetic retinopathy, and similar). We and many others have shown that these clinical diagnoses and classifications are subjective, and that there are often significant differences, even among experts, in making these diagnostic distinctions. This is a fundamental challenge that limits the accuracy and consistency of clinical ophthalmic diagnosis.
Anterior Segment and Artificial Intelligence
Damien Gatinel, Head of the Anterior Segment and Refractive Surgery Department, Rothschild Foundation Hospital, Paris, France
What are the differences between supervised and unsupervised learning in AI? Is the second option safe enough since we do not understand how the algorithm works?
In both cases, the prerequisite is identical; have a large amount of good quality data. In the case of supervised learning, we use labeled data, and we train an algorithm to classify data as inputs in the most efficient way or make predictions. In the pathology of the anterior segment, screening for keratoconus is an obvious application. To develop an effective algorithm, one must have training data from distinct groups (keratoconus versus normal cornea). Whatever the type of algorithm used (logistic regression, decision trees, neural networks), it will use input data for which its origin is clearly indicated. In the case of supervised learning, the approach is significantly different; the problem is usually to uncover hidden and unknown relationships present within a disparate dataset or to search for unknown patterns. It is less a question of predicting than discovering links between certain data that allow them to be grouped together, making it possible to classify large volumes of data. The algorithms reduce the dimensionality of the data entered in the system and estimate the distance in a smaller residual space between the data that one seeks to group. We used this process to evaluate the possibility of automatically classifying large volumes of topographic examinations, which can be of great interest for quickly finding specific categories (eyes operated on for refractive surgery, keratoconus, and similar). In any case, it is important to clear up confusion; if the algorithms are built according to a well-identified approach, the variables used to establish the groupings are not always easily identifiable. It is always necessary to be careful and have methods to limit the risk of overfitting and ensure that the system one develops remains generalizable.
The development of the PEARL-DGS Formula – the AI-based IOL calculation formula – is an important achievement. What are its parameters and how can it be used and tested today by practitioners?
The PEARL-DGS formula is based on an optical model using thick lens formulas, AI algorithms for the prediction of the anatomical position of the implant, and the curvature of the face posterior surface of the cornea (when this surface is not measured). The methods used correspond to supervised learning, made possible by obtaining a large set of quality data from pseudophakic eyes containing preoperative biometric data and the refractive result obtained. It also uses an axial length value per approximate or exact segment sum if the biometer provides this value. It makes it possible to consider a history of corneal refractive surgery and the results obtained for the first eye surgery to improve the precision of the power calculation. All the steps used to calculate the implant’s power have been published and the code was deposited in an online directory. The formula is available under the following link: www.iolsolver.com.
What will be the next AI-based applications in the anterior segment?
Anterior segment pathologies are a wide range of areas where one can consider using AI. The calculation of the implant’s power is of course already part of the landscape, but we are working on using neural networks and high-resolution OCT images of the anterior segment to establish an objective diagnosis of lens opacity. For a more objective distinction between proven cataracts (visually significant opacities) and non-dysfunctional lenses in terms of transparency. The same process has also been used to characterize the presence of corneal stromal edema. In both cases, it is possible to obtain an enriched image where the probability of lens opacity or corneal edema is shown for each pixel. Other interesting applications include improving the adaptation of contact lenses on irregular or reshaped corneas by quickly predicting the parameters of the lens, better predicting the size of phakic implants from biometric and refractive data. It is also possible to envisage diagnostic aid devices based on image banks of the anterior segment using conventional image recognition methods. Finally, we were interested in predicting subjective refraction from the objective measurement of the wavefront and high-degree optical aberrations. These are just examples, but the limits are those of your imagination!
- JH Wu, “Performances of machine learning in detecting glaucoma using fundus and retinal optical coherence tomography images: A meta-analysis,” Am J Ophthalmol, 237, 1 (2022). PMID: 34942113.
- AK Chaurasia, “Diagnostic accuracy of artificial intelligence in glaucoma screening and clinical practice,” J Glaucoma, 31, 285 (2022). PMID: 35302538.
- US Preventive Services Task Force et al., “Screening for primary open-angle glaucoma: US Preventive Services Task Force Recommendation Statement,” JAMA, 27, 1992 (2022). PMID: 35608574.
Andrzej Grzybowski is a professor of ophthalmology at the University of Warmia and Mazury, Olsztyn, Poland, and the Head of Institute for Research in Ophthalmology at the Foundation for Ophthalmology Development, Poznan, Poland. He is EVER President, Treasurer of the European Academy of Ophthalmology, and a member of the Academia Europea. He is a member of the International AI in Ophthalmology Society (https://iaisoc.com/) and has written a book on the subject that can be found here: link.springer.com/book/10.1007/978-3-030-78601-4.
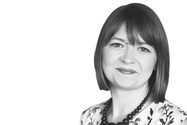
Having edited several technical publications over the last decade, I crossed paths with quite a few of Texere's current team members, and I only ever heard them sing the company's praises. When an opportunity arose to join Texere, I jumped at the chance! With a background in literature, I love the company's ethos of producing genuinely engaging content, and the fact that it is so well received by our readers makes it even more rewarding.