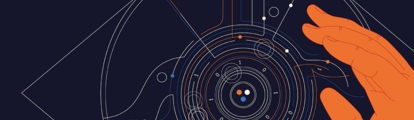
The Algorithms of Power: Part Three
Gurus of ophthalmic AI – Paisan Ruamviboonsuk and Michael D. Abràmoff – consider where and how the technology can help deliver the highest quality eye care
Andrzej Grzybowski, Aleksandra Jones | | 10 min read | Interview
Here, you can read Parts One and Two of this feature

Andrzej Grzybowski, Professor of Ophthalmology and Chair of Department of Ophthalmology, University of Warmia and Mazury, Olsztyn, Poland, and Head of Institute for Research in Ophthalmology, Foundation for Ophthalmology Development, Poznań, Poland
Our virtual AI in Ophthalmology Meeting in June 2022, sponsored by the Polish Ministry of Science and Education, turned out to be a great success, with over 600 registrations from over 20 countries, and lectures delivered by world-leading specialists in this field. I have received many requests to repeat the event next year.
As collaboration and networking of people interested in future applications of AI in ophthalmology is vitally important, I decided to start building the foundations for the International AI in Ophthalmology Society (IAIOph).
Everyone is welcome to join it directly at iaisoc.com or by emailing me: [email protected].
All the lectures from the 2022 AI in Ophthalmology Meeting are available at aiinophthalmology.com.
On Transfer Learning, GANs, and More
Paisan Ruamviboonsuk, Clinical Professor of Ophthalmology, College of Medicine, Rangsit University, Assistant Hospital Director for Centers of Medical Excellence, Center of Excellence for Vitreous and Retinal Disease, Rajavithi Hospital, Bangkok, Thailand
What is transfer learning and why do you think it can bring benefits to healthcare and ophthalmology?
Transfer learning (TL) is a type of deep learning model made use of other, already available, deep learning (DL) models or other datasets. TL may be used for the easier development of a DL model or to improve the accuracy of a DL model. For example, many models today were developed from information transferred from ImageNet, which is an open-source model available on the internet. In ophthalmology, information from OCT datasets, for example, can be transferred to corresponded datasets of color fundus images (CFI) to develop a DL model for making analysis on CFI, which may provide better accuracy than traditional DL developed from only data from CFI. This is because the model learns more from both CFI and OCT datasets. The benefits would include more AI models developed, with better performance.
What are the limitations of traditional AI models?
Traditional AI models may require a very large dataset developed to achieve high enough performance. In addition, there are a lot of data available today from multimodal imaging in ophthalmology, but traditional AI may be able to make use of only one type of data at a time.
What are GANs and how can they help ophthalmologists?
GANs is a DL model developed to create new images from existing images, and therefore, GANs is a TL model in nature. There are many uses of GANs in movie and advertisement industries, for example when creating an image of a zebra from an image of a horse. In medicine, GANs are used to create images of a modality that may be less commonly used, such as MRI, from images of a modality that may be more commonly used, such as a CAT scan. The new images may be used in AI research or to guide clinicians in clinical studies. In our study, we used GANs to create ultrasound biomicroscopy (UBM) images of anterior segment from corresponded anterior segment OCT images to detect plateau iris. In another study, researchers used GANs to create fundus images to unlock the black box of DL. The researchers in this study developed a DL model to detect where the nerve fiber layer or optic disc neuroretinal rim was thinning in fundus images of glaucomatous eyes. They used GANs to create a fundus image in which that thin area had normal thickness and another image in which that area was extremely thin. These new images highlighted where in fundus images the DL model used for making diagnosis of glaucoma and ophthalmologists could use these images created by GANs to judge if the model pointed out the correct areas.
AI: Autonomous or Assistive
Michael D. Abràmoff, The Robert C. Watzke, Professor of Ophthalmology, Professor of Electrical and Computer Engineering, and Biomedical Engineering, Department of Ophthalmology and Visual Sciences, University of Iowa Hospital and Clinics, Iowa, USA
What is the difference between autonomous and assistive AI medical devices?
The term “assistive” is for AI systems where the clinician makes the ultimate medical decision, and the clinician (the user) is liable for the AI performance, while the term “autonomous” is reserved for those systems where the AI makes the ultimate medical decision, and it is the AI creator who carries the liability for the AI performance, not the user. If someone claims autonomy for an AI, the next question should be whether the liability lies with the user (1).
Who should be responsible for a potential mistake made by an AI medical device?
Along with my colleagues, we previously proposed that creators of autonomous AI systems assume liability for harm caused by the diagnostic output of the device when used properly and on label (2). The article states that this is essential for adoption: it may be inappropriate for clinicians using an autonomous AI to make a clinical decision they are not comfortable making themselves, to nevertheless have full medical liability for harm caused by that autonomous AI. This view was recently endorsed by the American Medical Association in its 2019 AI Policy. Such a paradigm for responsibility is more complex for assistive AI, where medical liability may fall only on the provider using it, because they are ultimately responsible for the medical decision, or on a combination of both, where even the relative balance of liability of the AI user and the AI creator come into play (3).
What are the major concerns regarding AI and how can they be addressed?
All stakeholders in the healthcare system have valid concerns about AI that need to be addressed. Stakeholders include patients, patient organizations, physicians and other providers, bioethicists, medicolegal experts, regulators such as US FDA and US FTC and Joint Commission, and payers such as CMS (Medicare and Medicaid) and private payers. Is there patient or population benefit, such as outcome improvement, from the use of the AI? I have called AI that is technologically cool but offers no patient benefit “glamour AI.” Does it increase health disparities, or otherwise negatively affect some populations? Is there racial, ethnic or other bias in the safety or efficacy of the AI? Who is liable if something goes wrong? What happens with a patient’s data when AI is used, and how is patient data used in development and usage?
There may be other, not yet anticipated concerns out there. The only way to address these known and unknown concerns is with an ethical framework for AI, which starts with the basic millennia-old bioethical principles such as Autonomy, Justice, Beneficence and Non-maleficence, and Responsibility. By measuring how much a given AI system meets each of these bioethical principles, AI creators can build systems that address all concerns in a provable (falsifiable) manner; this is called metrics for ethics. I and others have published extensively on these subjects, including an ethical framework for AI that has itself been used to create regulatory consideration for AI with US FDA, and reimbursement considerations for US CMS and other payers, and these have all been applied successfully, leading to regulatory approval and reimbursement for autonomous AI in the US (1, 2, 3).
From the ethical framework, the following can be derived: AI technology also needs to be validated through a preregistered, peer reviewed clinical trial that is conducted in the intended clinical setting, with outcomes that meet or exceed all superiority endpoints. For example, IDx-DR exceeded all superiority endpoints at 87 percent sensitivity, 91 percent specificity, with a valid diagnostic result for 96 percent of subjects, and was proven to have no racial and ethnic bias all of which exceeds human specialist performance. These outcomes led to FDA clearance and helped establish trust with all industry stakeholders facilitating the adoption of autonomous AI into the Standards of Care for diabetes, reimbursement through CPT code 92229, and widespread system adoption. The ultimate goal of AI advancement is to improve patient outcomes by increasing access, lowering costs, and improving the quality of care that is available to the people who need it most.
What You Need to Know About AutoML…
By Tomasz Krzywicki, Data Scientist, Uniwersytet Warmińsko-Mazurski, Olsztyn, Poland
What is AutoML and how can it be used?
AutoML is a cloud computing software or service that aims to automatically produce predictive models that solve certain problems based on a transferred dataset. To use the AutoML tools, all we need is access to a computer with the appropriate software installed or access to the cloud computing that offers AutoML services. We should also remember to have a properly marked dataset for solving a given problem. The use of these tools is limited only to indicating the dataset and starting the process of finding optimal model architectures, which can be lengthy. With a bit of patience, we can use the created model in any form, for example deploy it in another server service or on any device, and analyze prediction metrics prepared with AutoML tools.
What are the available Auto ML types and how do they differ?
The most prominent division of AutoML tools is the computing environment. In the case of software installed on local computers, the calculations are performed on them. The analogy is with cloud computing services. It is worth noting that AutoML tools running on local computers may require vital hardware resources in the form of a graphics processor and a reasonable amount of operating memory, preferably at least 16 GB. This most apparent division is also related to the cost of these tools. AutoML software installed on local computers is mostly free, while cloud-based AutoML services come at a cost.
What is the cost of these ML devices?
The cost depends on factors such as the location of the server room where the computation is performed, the type and complexity of the problem being solved, the target location for model deployment, and the scale of computational resources used in the time spent on model creation. When planning the costs, the server space for storing the datasets should also be considered. Some services do not allow downloading the created models to the computer disk, but only deploy them in other server services, which involves additional costs. For example, one hour of the AutoML service running in Ohio, US, costs US$1 per node (virtual machine). However, cloud computing providers often offer a free trial period, which is enough to test the capabilities of AutoML services.
What are the major challenges to developing AI further in the near future?
Currently, the most popular method for creating intelligent systems is machine learning, which is a heuristic that involves fitting a mathematical function, or a group of mathematical functions, to a certain dataset to obtain an optimal solution in the form of predictions close to the labels in the dataset. Therefore, at present, artificial intelligence can learn certain patterns, but it cannot think, and it requires continuous monitoring. Some researchers believe that AI will soon come to the end of its development capabilities. Major players in the world of technology, however, are already researching an entirely new form of this field, taking inspiration from the human brain and, from my point of view, this is the biggest challenge for the near and more distant future.
Further Reading
- MD Abramoff et al., “Lessons Learned About Autonomous Ai: Finding a Safe, Efficacious, and Ethical Path through the Development Process,” Am J Ophthalmol, 214, 314 (2020).
- MD Abramoff et al., “A Reimbursement Framework for Artificial Intelligence in Healthcare,” NPJ Digit Med, 5 (2022). PMID: 35681002.
- MD Abràmoff et al., “Foundational Considerations for Artificial Intelligence Using Ophthalmic Images,” Ophthalmology, 129, e14 (2021). PMID: 34478784.
- MD Abramoff et al., “Diagnosing Diabetic Retinopathy with Artificial Intelligence: What Information Should Be Included to Ensure Ethical Informed Consent?” Front Med (Lausanne), 8 (2021). PMID: 34901083.
- DS Char et al., “Identifying Ethical Considerations for Machine Learning Healthcare Applications,” The American Journal of Bioethics, 20, 7 (2020).
Andrzej Grzybowski is a professor of ophthalmology at the University of Warmia and Mazury, Olsztyn, Poland, and the Head of Institute for Research in Ophthalmology at the Foundation for Ophthalmology Development, Poznan, Poland. He is EVER President, Treasurer of the European Academy of Ophthalmology, and a member of the Academia Europea. He is a member of the International AI in Ophthalmology Society (https://iaisoc.com/) and has written a book on the subject that can be found here: link.springer.com/book/10.1007/978-3-030-78601-4.
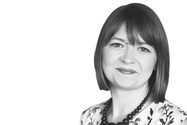
Having edited several technical publications over the last decade, I crossed paths with quite a few of Texere's current team members, and I only ever heard them sing the company's praises. When an opportunity arose to join Texere, I jumped at the chance! With a background in literature, I love the company's ethos of producing genuinely engaging content, and the fact that it is so well received by our readers makes it even more rewarding.