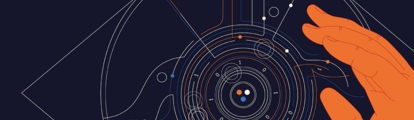
The Algorithms of Power: Part One
Gurus of ophthalmic AI celebrate the development of artificial intelligence technologies and presents questions to be addressed before the AI-based medical devices are introduced to real life
Andrzej Grzybowski, Aleksandra Jones | | 9 min read | Interview
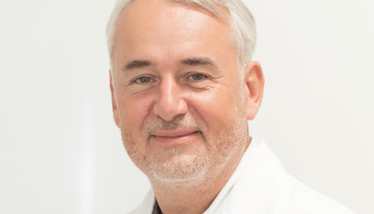
Hazards and Potential Problems of AI Medical Devices
By Andrzej Grzybowski, Professor of Ophthalmology and Chair of Department of Ophthalmology, University of Warmia and Mazury, Olsztyn, Poland, and Head of Institute for Research in Ophthalmology, Foundation for Ophthalmology Development, Poznań, Poland
We have recently seen a significant development of many AI-related technologies and applications, and there’s a lot of enthusiasm about the promises of AI in healthcare, including improving patient and population outcomes, making medical teams’ work easier, as well as reducing costs by avoiding errors and unnecessary procedures. We have entered the fourth stage of the Industrial Revolution and AI is its most important theme.
Ambitious expectations for AI in healthcare include outperforming doctors, helping to diagnose what is presently undiagnosable to treat what is currently untreatable, predicting the unpredictable, and classifying the unclassifiable. AI might help preserve the doctor-patient relationship and move it from the present “shallow medicine” into “deep medicine” based on deep empathy and connection. Currently, the average time of a clinic visit in the US for an established patient is seven minutes, and for a new patient – 12 minutes, and in many Asian countries it is down to two minutes per patient. To make this even worse, part of this time must be devoted to completing the electronic health record.
AI-based “deep medicine” might give us more time for crucial relations with our patients – and those cannot be replaced by technology. AI-based technologies using the deep-learning (DL) approach have been shown to support decisions in many medical specialties, including radiology, cardiology, oncology, dermatology, and ophthalmology. AI/DL models have reduced waiting times, improved medication adherence, customized insulin dosages, and helped interpret magnetic resonance images. AI/DL algorithms were shown to detect disease states based on image analysis, including retinal diseases from fundus photos and OCT scans, lung diseases from chest radiographs, and skin disorders from skin photos. Two autonomous AI-based medical devices are registered in the US for detection of diabetic retinopathy, and a few more are available in the EU; AI algorithms have been used for DR screening in many parts of the world.
In Poland, I started an AI-based DR screening project in 2017, and since 2018, my team has been conducting a big project aiming to screen 40,000 diabetic patients in the Wielkopolska region, funded by the EU. A new and very promising application is to use eye images to identify risk of cardiovascular or neurodegenerative disorders.
However, when talking about the increasing enthusiasm around and prospects of AI in ophthalmology, we must also mention rising problems and questions that need to be addressed before the AI-based medical devices are introduced to real life.
One of the main problems is the lack of clarity of what constitutes the evidence of impact and demonstrable benefit for the many AI-medical devices, and who can assess the evidence.
The future development of the AI field depends on an easier – and, preferably, unlimited – access to the medical data stored within the electronic health records. This access, however, cannot constitute privacy overuse of this very sensitive data. According to the US National Institute of Standard and Technology, biometric data, including retina images, are personally identifiable information and should be protected from inappropriate access.
Although present AI models were shown to diagnose and stage some ocular diseases from images, including fundus photos, OCT and visual field data, most AI algorithms were tested on a dataset not corresponding well to real-life conditions. Patient populations were usually homogeneous regarding ethnicity, age, lack of comorbidities, and poor-quality images.
Moreover, some algorithms were shown to misrepresent and exacerbate health problems in minority groups. Future datasets should better describe who is represented and in what way, to avoid structural biases (please see one of the recent initiatives at www.datadiversity.org).
Thus, future studies on validating algorithms on real life ocular images from heterogenous populations are needed, including both good- and poor-quality images. Otherwise, we may face a “good-AI-gone-bad.” Cherry-picking best results might make the situation even worse. It should be highlighted that AI-based algorithms might behave unpredictably when applied in the real world. It has been shown that performance of the algorithm degrades when applied to images generated by a different device or in a different clinical environment to those of the training set. All these problems might lead to misdiagnosis and erroneous treatment suggestions, breaching the trust in AI technologies. Finally, we should be able to imagine that if an AI system made an error, it could harm hundreds or even thousands of patients. Thus, I like to repeat Tetlock & Gardner (Superforecasting) who said: “If you do not get feedback, your confidence grows much faster than your accuracy.”
One of the recent independent studies comparing seven different algorithms, reported that one of the tested algorithms was significantly worse than human graders at all levels of DR severity – it missed 25.58 percent of advanced retinopathy cases, which could potentially lead to serious consequences (1). This study showed possible problems and patient safety hazards related to clinical use of some algorithms. They include limitations related to training of an algorithm on particular demographic group, including ethnicity, age, sex, and its further use on a different population. Moreover, many studies exclude low-quality images, treated as ungradable images, and patients with comorbid eye diseases, which makes them less reflecting the conditions of real life.
It should be also remembered that AI algorithms can be designed to perform in unethical ways. For example, Uber’s software, Greyball, was designed to allow the company to identify and circumvent local regulations and Volkswagen’s algorithm that allowed vehicles to pass emission tests by reducing their nitrogen oxide emission when they were being tested. Moreover, clinical decision-support algorithms could be designed to generate increased profits for their owners, such as recommending particular drugs, tests, and more, without clinical users’ awareness. Finally, AI systems are vulnerable to cybersecurity attacks that could cause the algorithm to misclassify medical information. For more on this subject, reach for our recent publication, Artificial Intelligence in Ophthalmology (2).

Our virtual AI in Ophthalmology Meeting in June 2022, sponsored by the Polish Ministry of Science and Education, turned out to be a great success, with over 600 registrations from over 20 countries, and lectures delivered by world-leading specialists in this field. I have received many requests to repeat the event next year.
As collaboration and networking of people interested in future applications of AI in ophthalmology is vitally important, I decided to start building the foundations for the International AI in Ophthalmology Society (IAIOph).
Everyone is welcome to join it directly at iaisoc.com or by emailing me: [email protected].
All the lectures from the 2022 AI in Ophthalmology Meeting are available at aiinophthalmology.com.
What are the major challenges to developing AI further in the near future?
Linda Zangwill, Professor of Ophthalmology in Residence, Richard K. Lansche and Tatiana A. Lansche Endowed Chair, Co-Director of Clinical Research, Hamilton Glaucoma Center Director, Data Coordinating Center, Shiley Eye Institute, UC San Diego, California, USA
Development of AI algorithms to detect glaucoma is now relatively straightforward if one has appropriate datasets and computational resources. One of the major challenges to the implementation of AI in clinical settings is to ensure that the algorithm is generalizable to the targeted populations and not biased due to limitations of the training set. Evaluating the generalizability of the results requires extensive testing of the AI algorithm on external datasets from diverse populations. Another challenge is determining how to integrate the AI system and results into clinical practice. Where and how should the AI algorithm results be placed in the electronic health record or PACS system that the clinician uses in their routine management of glaucoma patients? What type of summary information and/or visualization of the AI results should be provided? It is essential to determine how the AI results can be provided in a way that is easy and fast to use so that it provides added value and does not slow down the busy clinical workflow. One can develop the best AI algorithm, but if clinicians are not willing or able to use it, it will not improve clinical care. Other challenges for the development and implementation of AI include how best to open the black box to provide information on what the algorithm used to make its decision, as well as medical, legal, ethical, and privacy issues.
Michael F. Chiang, Director, National Eye Institute, National Institutes of Health, Bethesda, Maryland, USA
I will articulate a few challenges: first, we are losing many opportunities to utilize ophthalmic image data for developing AI systems because those data are locked in proprietary standards and inaccessible to researchers and clinicians. Second, we need to improve the culture of data sharing, standards for data representation, and methods for establishing ground truth to take full advantage of building large, AI-ready datasets for knowledge discovery. Third, AI systems are best at addressing discrete questions (such as “Is there plus disease in this retinal image from a baby undergoing ROP screening?”), whereas real-world scenarios require addressing numerous questions in parallel. Fourth, AI systems are typically trained and validated in fairly narrow populations and specific imaging devices, whereas real-world applications will need to be rigorously validated to ensure they work across broad populations and devices without bias.
Damien Gatinel, Head of the Anterior Segment and Refractive Surgery Department, Rothschild Foundation Hospital, Paris, France
The limits of AI development mainly concern data collection because the common point of any project is to use a large volume of quality data. It is common that even when a large data set has been compiled, it is necessary to reduce its size drastically.
We can also foresee certain ethical problems insofar as we sometimes do not know by what mechanism(s) certain results are obtained in terms of classification or prediction.
Paisan Ruamviboonsuk, Clinical Professor of Ophthalmology, College of Medicine, Rangsit University, Assistant Hospital Director for Centers of Medical Excellence
Center of Excellence for Vitreous and Retinal Disease, Rajavithi Hospital, Bangkok, Thailand
I think we can take advantage of multimodal images in ophthalmology to develop AI models that are more efficient in screening or detecting diseases or detecting disease progression. There are countless AI models for different kinds of tasks today; however, the major challenges for me rest on how useful these models are in reducing the risk of blindness; how useful they are to be deployed in the real-world. Many AI models work well in internal validation but fall short in real-world deployment. The other challenges would rest on the “prediction” of treatment outcome and disease progression. The models for these tasks now have accuracy around 70 percent, we look forward to better predictions in the future.
Michael D. Abràmoff, The Robert C. Watzke, Professor of Ophthalmology, Professor of Electrical and Computer Engineering, and Biomedical Engineering, Department of Ophthalmology and Visual Sciences, University of Iowa Hospital and Clinics, Iowa, USA
Theoretical challenges that I see: in healthcare, training data will always be sparse, so how can we build AIs that use limited amounts of training data and how do we use proxies under deep learning conditions? Under what conditions can an AI be changed “somewhat” without requiring full (and often expensive) validation? We have to be able to figure out how we expand reimbursement for AIs that meet some but not all of the criteria above, and how we deal with the information loss that comes with repeated examination of existing datasets, such as an expensive validation dataset. Practical challenges that I predict include, but are not limited to: the need for better education and adoption of highly validated AI systems that are integrated into clinical workflows and sustainably reimbursed. AI in healthcare needs to focus on solutions that offer the greatest benefit to patients. How do we regulate vernacular AIs that are safe and effective in certain subpopulations but not others? While there may be AI technologies that sound exciting, if they aren’t positively impacting patient outcomes, they won’t bring any real benefit to healthcare and could slow the adoption of the solutions having a positive impact. Of course, all of this is dependent on having access to appropriately diverse and reliable data sets with which to train new AI systems.
- AY Lee et al., “Multicenter, head-to-head, real-world validation study of seven automated artificial intelligence diabetic retinopathy screening systems,” Diabetes Care, 44, 1168 (2021). PMID: 33402366.
- A. Grzybowski (ed), Artificial Intelligence in Ophthalmology. Springer: 2021. https://link.springer.com/book/10.1007/978-3-030-78601-4.
Andrzej Grzybowski is a professor of ophthalmology at the University of Warmia and Mazury, Olsztyn, Poland, and the Head of Institute for Research in Ophthalmology at the Foundation for Ophthalmology Development, Poznan, Poland. He is EVER President, Treasurer of the European Academy of Ophthalmology, and a member of the Academia Europea. He is a member of the International AI in Ophthalmology Society (https://iaisoc.com/) and has written a book on the subject that can be found here: link.springer.com/book/10.1007/978-3-030-78601-4.
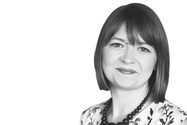
Having edited several technical publications over the last decade, I crossed paths with quite a few of Texere's current team members, and I only ever heard them sing the company's praises. When an opportunity arose to join Texere, I jumped at the chance! With a background in literature, I love the company's ethos of producing genuinely engaging content, and the fact that it is so well received by our readers makes it even more rewarding.