State of the Art(ificial)
There is almost endless potential to AI in ophthalmology, but it will never replace human judgement
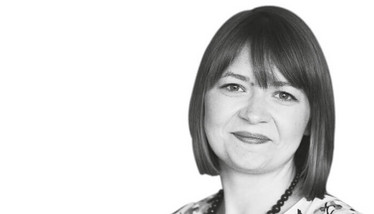
The application of Artificial Intelligence (AI) in ophthalmology has been a hot topic for the last few years, it has recently been gaining serious traction. From detecting retinal diseases to identifying existing drugs that can help reduce vision loss associated with age-related macular degeneration (1), the possibilities appear almost endless. Algorithms have the potential to cover much of the spectrum of the clinician’s practice: from diagnosis to treatment decisions to compliance monitoring.
Prevalence and risk factors can already be evaluated much more efficiently (2); and deep learning is not only being used to improve healthcare in developed countries – but it is also helping populations with inadequate access to eye care. Take the cutting-edge technology helping to screen thousands of people in Africa for diabetic retinopathy, for example (3).
Pearse Keane, consultant ophthalmologist at Moorfields Eye Hospital in London, UK, said that “ophthalmology will be the first field transformed by AI.” The staggering 94 percent correct referral rate for 50 different retinal disorders seems to confirm his words (4). AI used to aid detection and diagnosis not only takes a lot of the guesswork out of the equation, it is also ultimately objective – something that humans, including physicians, have always struggled with.
In this issue’s feature Raymond Radford asserts that unconscious bias is inherently present in glaucoma care, and is responsible, among other aspects, for a widespread variability in assessing CDR or deciding on the acceptable IOP levels. The impact of information bias, overconfidence or risk tolerance has been associated with diagnostic inaccuracies in up to 77 percent of cases of physicians making medical decisions (5).
However, as Chelvin Sng and Dan Lindfield point out in our feature, there are vital aspects of diagnosing and managing glaucoma that it is hard to imagine a machine could learn: intuition, albeit based on training and expertise, and the ability to understand the patient’s – often irrational – behaviour and preferences.
AI is moving along quickly in the field of retinal conditions. Its proponents are quick to show its efficacy in various other areas of ophthalmology, but we might have to wait a little longer to see the difference it will make in glaucoma management. If it can minimize the human error, and complement ophthalmologists’ existing practice, it will definitely be worth the wait (6).
Aleksandra Jones
Editor
- “Shake-up in AI drug discovery”, Nat Biotechnol, 37 (2019). PMID: 31164750.
- D Ting et al., “Deep learning in estimating prevalence and systemic risk factors for diabetic retinopathy: a multi-ethnic study”, NPJ Digital Medicine, 2 (2019). DOI: 10.1038/s41746-019-0097.
- V Bellemo et al., “Artificial intelligence using deep learning to screen for referable and vision-threatening diabetic retinopathy in Africa: a clinical validation”, Lancet Digital Health, 1, 35 (2019). DOI: 10.1016/S2589-7500(19)30004-4.
- J De Fauw et al., “Clinically applicable deep learning for diagnosis and referral in retinal disease”, Nat Med, 24, 1342 (2018). PMID: 30104768.
- G Saposnik et al., “Cognitive biases associated with medical decisions: a systematic review”, BMC Med Inform Decis Mak, 16, 138 (2016). PMID: 27809908.
- C Zheng et al., “Artificial intelligence in glaucoma”, Curr Opin Ophthalmol, 39, 97 (2019). PMID: 30562242.
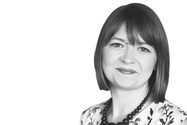
Having edited several technical publications over the last decade, I crossed paths with quite a few of Texere's current team members, and I only ever heard them sing the company's praises. When an opportunity arose to join Texere, I jumped at the chance! With a background in literature, I love the company's ethos of producing genuinely engaging content, and the fact that it is so well received by our readers makes it even more rewarding.