Retinal Imaging: The Big Picture
Highlights from the “Utilizing Ultra-Widefield Retinal Imaging – Clinical Applications and Practice Efficiency” satellite meeting held at the 17th EURETINA Congress, Barcelona, on Friday 8th September 2017, which featured presentations from Paulo Stanga (Chair), Eric Souied, Antonia Joussen, Sobha Sivaprasad and SriniVas Sadda.
sponsored by Optos
Retinal imaging has come a long way since the first ophthalmic photographs were taken in 1886 by Jackman and Webster. The first commercially-available fundus camera had a 20° field of view; today we have digital ultra-widefield (UWF) technology that produces 200° images in less than half a second. This UWF view is profoundly changing how we diagnose and manage retinal disease – particularly patients with diabetic retinopathy (DR). But there is a problem – inertia.
Today’s fundus cameras typically capture between 30° to 40° of the retina – you can effectively image the macula, but you miss the periphery. Using this technology, multiple images, montaged together, can show more of the retina, but these montages fall far short of the equator and far periphery. The seminal Diabetic Retinopathy Study, published in 1991 (1) introduced the 7-Standard Field (7SF) approach (Figure 1). This has been the standard ever since, and a great deal of clinical data has been accumulated using it. However, current technology allows us (and even compels us) to do better.
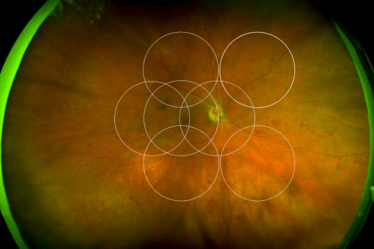
Figure 1. A 200° Optomap UWF image with the 7-standard field superimposed upon it.
Let’s take the example of DR. Traditional, “limited field” fluorescein angiography (FA) transformed the diagnosis and management of DR by revealing microaneurysms, non-perfusion, neovascularization and macular edema. In recent years, research with UWF FA has proven that much of this pathology – particularly non-perfusion – presents first in the periphery. These studies also show that much of this pathology is missed by 7SF imaging (2). Kaines et al. (3) found that when they laid a 7SF template on to 200° UWF FA images (Figure 1), UWF FA revealed:
- 3.2 times the amount of retinal area,
- 3.9 times the amount of retinal non-perfusion,
- 1.9 times the area of neovascularization, and
- 3.8 times the area of panretinal photocoagulation (PRP) than the simulated 7SF image.
Silva et al. (4) showed that non-perfusion areas at the mid- and far-periphery detected by UWF imaging accounted for ~86 percent of the total ischemic area. Even more significantly, in 10 percent of cases, peripheral lesions seen in UWF images resulted in a more severe DR assessment than when patients were assessed only with EDTRS 7SF imaging (5). This shows that the information provided by peripheral imaging is not just interesting, it can be crucial for proper clinical assessment.
There’s another problem with 7SF imaging in terms of DR staging scales (6), in that its precision is limited: it’s just not quantitative. We live in an age of anti-VEGF therapy that can treat the underlying retinopathy and we regularly see multiple levels of improvement. But DR staging scales are truly insufficient to characterize what’s happened to the retina – and this is particularly concerning as there are a number of Phase III trials still using this two-step improvement as an endpoint. Furthermore, it’s unclear whether the existing system is clinically practical because many clinicians now just refer to “background retinopathy.” The biggest question: is this actually based on enough retina when there’s so much retinal territory beyond the seven standard fields?
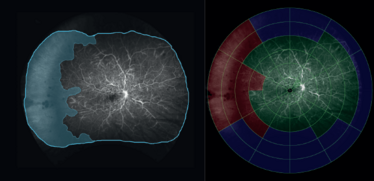
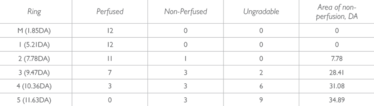
Figure 2. An example of the concentric rings retinal topography non-perfusion grading assessment, M0 10 21 33 43 53. The green cells and the red cells represent the perfused and non-perfused regions respectively. The results are tabulated and the area easily determined. The results can be written as a subscript for cells graded as non-perfused thus giving the reader an appreciation of the area and distribution of ischemia.
So how can UWF imaging be leveraged to improve care?
Retinal vein occlusions (RVOs) are typically classified on 7SF – if it’s more than 10 disk areas, it’s an ischemic central RVO; if it’s more than five, it’s an ischemic branch RVO, and macular ischemia (and its response to treatment) tends to be characterized by measuring the change in foveal avascular zone size. But if we see additional non-perfusion in the periphery with UWF imaging, we often have to change our classification. Clearly, a better grading method is necessary.
UWF imaging enables a more objective approach. For example, the “ischemic index” is a widely used measure of non-perfusion in UWF FA. To calculate the ischemic index, the area of non-perfused retina is divided by the area of gradable retina. This approach has improved the prognostication of retinal disease; Tsui et al. (7) found that an ischemic index of 45 percent or greater was associated with an increased probability of the patient developing neovascular complications. The drawback to this approach is care has to be taken to correct for 3D to 2D projection errors, and the fact that the periphery is magnified in these images. If not correctly accounted for these projection errors will exaggerate peripheral ischemia. A more detailed and descriptive approach to topographically measuring retinal non-perfusion in UWF FA is the 6 concentric ring approach (Figure 2). The concentric rings consists of 6 rings with 12 cells in each ring. There the central (macula) ring is defined as Ring M, and Rings 1-5 surround it. Each cell is graded as perfused (if ≥50 percent of the ring appears perfused), not perfused (if <50 percent of the ring displays perfusion) or ungradable. Each cell in each ring corresponds to a pre-calculated number of disk areas in size for example, each cell in Ring M is 2 DA, and each in Ring 1, 6.3 DA. One study compared the concentric ring approach with the ischemic index method of assessing retinal ischemia in UWF angiograms from 28 eyes with central RVO (8). Five graders assessed the images using both methods, and it was found that the inter-test agreement between concentric rings and the ischemic index method using the intra-class correlation coefficient was 0.965. Inter-grader agreement was 0.910 for the concentric rings method and 0.898 with the ischemic index method. The test-retest reliability was 0.975 for the rings and 0.979 for the ischemic index. Average grading time per image was 187 s and 297 s for the concentric rings method and ischemic index method, respectively, p<0.001. In other words, the 6 concentric ring approach has “an ‘almost-perfect’ inter-grader and inter-test agreement with the ischemic index method” – with a shorter grading time (8).
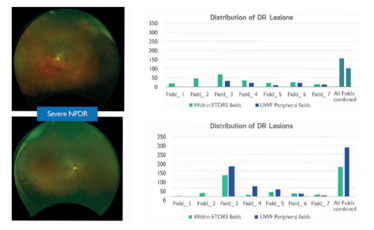
Figure 3a. Additive information from peripheral assessment: predominantly central (above) vs. predominantly peripheral (below) profiles for same ETDRS level of retinopathy.
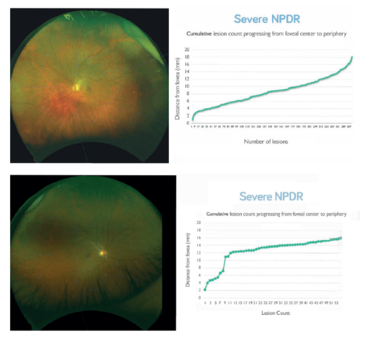
Figure 3b. Different severe non-proliferative diabetic retinopathy (NPDR) profiles revealed by cumulative lesion count as a function of distance from the foveal center.
But the next step towards a truly objective assessment of retinal pathology is a combination of UWF digital imaging and automated image processing. There are a number of CE-marked, automated lesion detection products on the market which use standard retinal imaging, and Tufail et al. (9) found that some of these systems provide acceptable sensitivity for referable retinopathy when compared with that of human graders – and had sufficient specificity to make them cost-effective alternatives to manual grading alone. Can this approach also work with UWF imaging? A pilot study, using as-yet unoptimized for UWF lesion detection algorithms, is already showing similar screening abilities to the non-widefield approaches (10). However, the power of this approach is that it provides quantitative lesion count maps – and can plot the lesions by location (Figure 3a), or even as a cumulative lesion count that progresses from the center to the periphery (Figure 3b). It enables you to view different phenotypes of disease based on these profiles – and may help us further segregate these different presentations of DR.
What’s next is an agnostic, artificial intelligence-based approach, where a deep-learning neural network determines what features it should use to, for example, distinguish patients who will progress to proliferative DR (PDR). In essence: you show the computer images of retinas that did progress to PDR, and those which didn’t. Eventually, you can show the computer a retinal image and it can say: yes, this patient will progress with a high certainty – and there are already deep learning algorithms that can detect referable DR with 97.5 percent sensitivity and 93.4 specificity (11).
In summary, peripheral lesions are important: if you see more, you know more, and UWF imaging has become the standard for staging DR. Artificial intelligence is going to transform how easily and quickly we can perform automated image analysis and transform the quantitative scoring of diabetic retinal disease. The adoption of this will have huge practical and efficiency implications: rapid, UWF digital imaging speeds patient throughput; automated image analysis will save considerable amounts of time and resource, meaning that treatment decisions can be made quickly, based on better, more comprehensive evidence. Between UWF imaging and artificial intelligence, the future will be one of seeing more – and treating more in the most efficient way possible.
- Early Treatment Diabetic Retinopathy Study Research Group, “Fundus photographic risk factors for progression of diabetic retinopathy. ETDRS report number 12”, Ophthalmology, 98, 823–833 (1991). PMID: 2062515.
- MM Wessel et al., “Ultra-wide-field angiography improves the detection and classification of diabetic retinopathy”, Retina, 32, 785–791 (2012). PMID: 22080911.
- A Kaines et al., “Ultrawide angle angiography for the detection and management of diabetic retinopathy”, Int Ophthalmol Clin., 49, 53–59 (2009). PMID: 19349786.
- PS Silva et al., “Diabetic retinopathy severity and peripheral lesions are associated with nonperfusion on ultrawide field angiography”, Ophthalmology, 122, 2465–2472 (2015). PMID: 26350546.
- PS Silva et al., “Peripheral lesions identified by mydriatic ultrawide field imaging: distribution and potential impact on diabetic retinopathy severity”, Ophthalmology, 120, 2587–2595 (2013). PMID: 23778092.
- CP Wilkinson et al., “Proposed international clinical diabetic retinopathy and diabetic macular edema disease severity scales”, Ophthalmology, 110, 1677–1682 (2003). PMID: 13129861.
- I Tsui et al., “Reliability of ischemic index grading in common retinal vascular diseases”, Ophthalmic Surg Lasers Imaging Retina, 46, 618–625 (2015). PMID: 26114841.
- L Nicholson et al., “Validation of concentric rings method as a topographic measure of retinal nonperfusion in ultra-widefield fluorescein angiography”, Am J Ophthalmol., 160, 1217–1225 (2015). PMID: 26384165.
- A Tufail et al., “Automated diabetic retinopathy image assessment software: diagnostic accuracy and cost-effectiveness compared with human graders”, Ophthalmology, 124, 343–351 (2017). PMID: 28024825.
- K Wang et al., “Automated detection of diabetic retinopathy lesions on ultrawidefield pseudocolour images”, Acta Ophthalmol, [Epub Ahead of Print] (2017). PMID: 28926199.
- V Gulshan et al., “Development and validation of a deep learning algorithm for detection of diabetic retinopathy in retinal fundus photographs”, JAMA, 316, 2402–2410 (2016). PMID: 27898976.