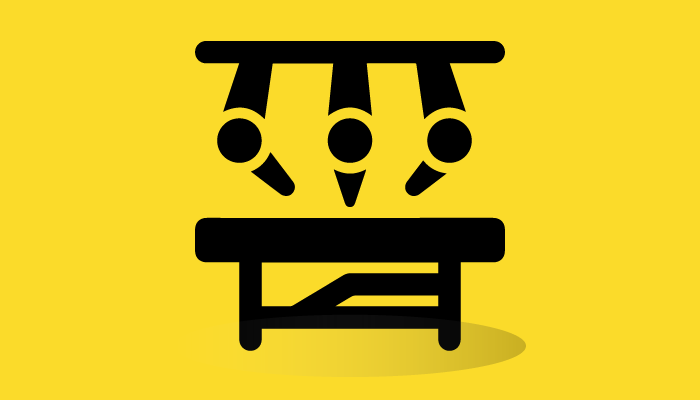
AI has come to the forefront of public consciousness in the past few years. From organizing photos on smartphones to self-driving software in automobiles, new applications for AI are discovered every day – especially in the context of healthcare. I believe the potential of AI to refine the precision of surgical devices, enhance surgical efficiency, and improve patient outcomes is truly being realized today.
I recently had the opportunity to delve into the deep learning being done to incorporate AI into femtosecond laser-assisted cataract surgery (FLACS), particularly for diagnostic image segmentation and optimizing fragmentation patterns during cataract surgery. The following is a summary of research recently presented at ARVO and a look at where deep learning, AI, FLACS, and robotic cataract surgery may be headed in the future.
Diagnostic image segmentation
Cornea and lens segmentation. One of the most impressive applications of deep learning in FLACS is the segmentation of Scheimpflug images to precisely identify the boundaries of the cornea and lens, a crucial step in accurately planning and executing cataract surgery.
In a study presented at ARVO, researchers analyzed 973 eyes with AI to delineate these boundaries with remarkable accuracy (1). About 220 eyes were from surgeries performed with the LENSAR Laser System (LENSAR) in a commercial setting, and the remaining eyes were imaged by ALLY (LENSAR) in the following settings: commercial surgeries (n = 628), a clinical study environment (n = 64), precommercial clinical data collection activities of noncataracts (n = 31), and ex vivo scans obtained in a laboratory setting (n = 28).
The researchers manually delineated the boundaries of the cornea and the lens surfaces and performed aggressive data augmentation including illumination changes, noise addition, affine transformations, and histogram stretching to train a deep neural network (DNN) of Scheimpflug images to identify pixels of the anterior and posterior surfaces of the lens capsule and cornea.
All Scheimpflug images included in the study, regardless of the setting, showed delineation of the anterior surface of both the cornea and lens with zero failures to reconstruct these surfaces. However, reconstruction of the posterior surface of the lens failed in five eyes. In three instances, the density of the cataract was too great and obscured accurate identification of the posterior lens. All successful reconstructions were screened for visible surface detection errors. Only one and eight cases of visible surface detection errors were found for the anterior and posterior lens surfaces, respectively. The DNN output produced a usable 3D lens reconstruction and one free discernible error in 99.7 percent and 98.8 percent of cases, respectively.
The researchers concluded that this DNN can be used to repeatedly and accurately identify the boundaries of the cornea and lens capsule at a pixelar level, even in the presence of dense cataracts where the posterior lens capsule is difficult to visualize (Figure 1).
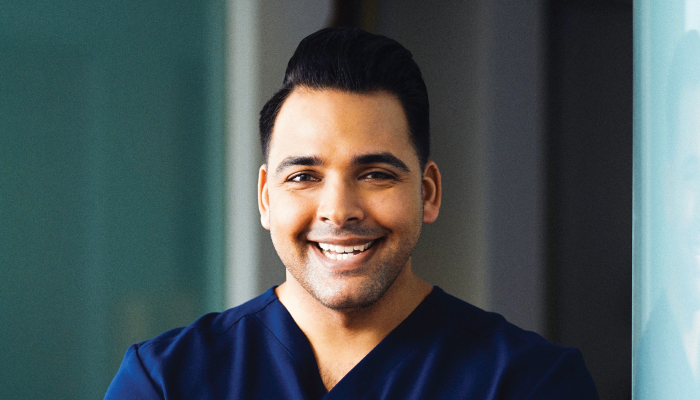
Arjan Hura
Pupil, limbus, and eyelid segmentation. A separate yet related study presented at ARVO showed that a DNN can also be used to segment iris images and identify the pupil, limbus, and eyelid boundaries from various topography devices with a unified software approach (2). Researchers manually delineated the boundaries of the pupil and visible iris of 604 grayscale topography images and applied a custom pupil warping method to produce augmented copies of each image with the iris manipulated to varying pupil shapes and sizes (Figure 2). A DNN was then trained to label pixels as from the pupil, iris, or neither.
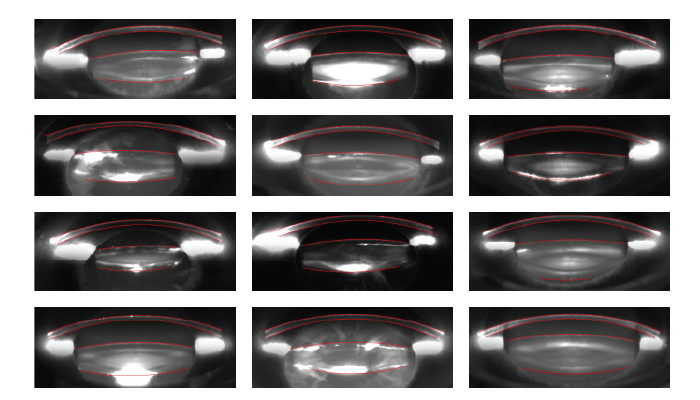
Figure 1. Sampling of successful segmentations for various cataract morphologies. (Courtesy of Dustin Morley and Mike Evans.)
Accurate identification of the pupil and limbus was achieved in all but one eye, and the pupil center error was fewer than 5, 10, and 15 pixels in 98.7 percent 99.5 percent, and 99.7 percent of eyes, respectively. Similarly, the limbus center error was fewer than 5, 10, and 15 pixels in 69.2 percent, 95 percent, and 99.8 percent of eyes, respectively. Dice coefficients of greater than 0.95 was achieved in 95.6 percent, 98.2 percent, and 92 percent of pupil region, composite iris region, and exclusive iris region, respectively.
Clinical applications. The clinical applications of these two related studies are significant. Improving the accuracy of 3D reconstructions of the cornea and lens enhances the precision of FLACS, which has been shown to result in a reduction of overall intraoperative case time. This increased accuracy has also been shown to reduce phacoemulsification time for FLACS users, leading to the use of less intraoperative phacoemulsification energy, possibly less corneal edema, and thus, a potential improvement in immediate surgical outcomes and faster postoperative recovery and visual acuity for patients. Additionally, these technological advances can improve device interoperability and enable software to process images from multiple diagnostic devices with little or no modification for iris registration and identification of potential for cyclotorsion when treating astigmatism either with astigmatic keratotomy or guiding toric IOL alignment.
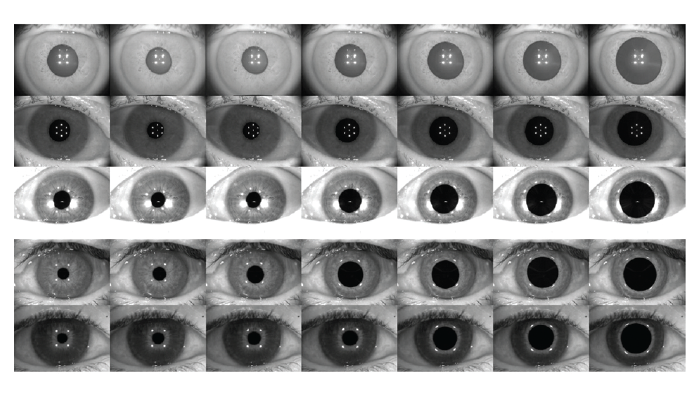
Figure 2. The original topography images were manipulated to create various pupil shapes and sizes. (Courtesy of Dustin Morley and Mike Evans.)
Optimizing fragment patterns
Another exciting development is the application of AI to optimize fragmentation patterns. During a recent LENSAR user meeting, Jonathan Solomon shared his experience working with the company to refine his custom laser fragmentation patterns. Analyzing his baseline settings and then using AI to adjust parameters such as spot spacing led to better cleavage and increased his pattern from a two-plane to a three-plane chop plane. This helped Solomon achieve a 20 percent reduction in his overall case time and decreased the amount of phaco energy he used in routine cases. As with AI-based Scheimpflug image segmentation, the clinical implication of optimizing fragmentation patterns with deep learning is meaningful, including a shorter surgical time, a reduced risk of intra- and postoperative inflammation, faster recovery, and better postoperative day one visual outcomes.
Even for users of this femtosecond laser technology who may not have the opportunity to work directly with the manufacturer to refine their personal fragmentation patterns, the benefits of these advancements are very accessible. The data and improvements generated from the collaborative efforts of researchers and key clinicians are integrated into the next-generation models of DNNs, resulting in software upgrades that benefit all those who use the technology in clinical practice. This democratization of advanced surgical techniques ensures that we can all leverage innovations to improve our practice and patient outcomes, especially in complex cases like dense cataracts and eyes with zonulopathy.
Forward progress
Looking ahead, the integration of AI and deep learning into FLACS paves the way for the next frontier – robotic cataract surgery. This potential future will be driven by sophisticated AI algorithms capable of processing big data to perform precise surgical tasks. While we are still in the early stages of robotic cataract surgery, the progress made by the researchers working on these developments is a crucial step toward making robotic cataract surgery a reality.
Incorporating AI and deep learning into FLACS underscores the transformative shift currently happening in our field. Further developments should enhance the precision of surgical innovations, optimize our surgical techniques, streamline our workflow, and ultimately improve patient outcomes. As we continue to embrace AI in ophthalmology, we are not only advancing our current practice but also laying the groundwork for the future of cataract surgery.
It is an exciting time to be in this field.