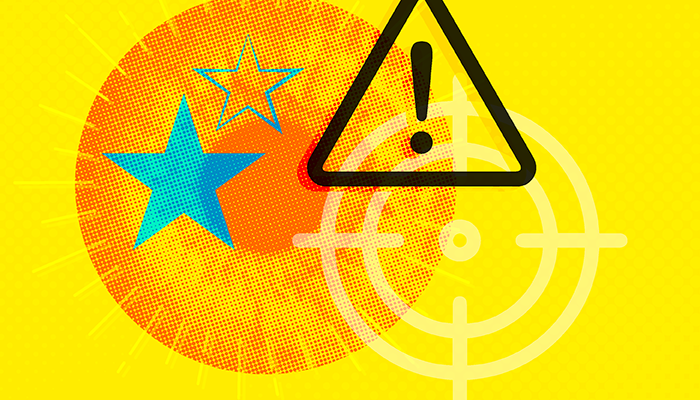
How can we improve early risk stratification in glaucoma? While there are a number of viable answers to this question, I see deep language models (DLM) as a particularly promising approach. By 2040, estimates suggest there will be more than 110 million people affected by the condition worldwide – though, if appropriately treated, only a small number of these patients will undergo significant visual deterioration over time.
Identifying and treating patients at higher risk for rapid glaucoma worsening earlier is paramount for reducing the possibility of vision loss and functional impairment. Specifically, early risk stratification enables non-specialists, like general ophthalmologists and optometrists, to refer higher-risk patients to glaucoma specialists promptly while setting longer follow-up intervals for lower-risk individuals. There’s just one problem: we’re short-staffed.
Though the number of eye care professionals in most countries has increased in recent years, it still remains insufficient (1). Indeed, we are at the precipice of a surge in glaucoma cases and relying solely on fellowship-trained glaucoma specialists to triage all patients may become impractical. DLMs, in this regard, could be key. In fact, we recently trained a DLM using data from glaucoma patients at the Wilmer Eye Institute spanning 2013 to 2021 (2) – a process in which we sought to predict which eyes would go on to need surgery for uncontrolled glaucoma.
Our dataset comprised several thousand eyes with baseline visual field (VF) and optical coherence tomography (OCT) data, as well as clinical and demographic information. During preprocessing, we oriented and stacked OCT and VF data into a three-channel image. Then, a vision transformer was used to extract features from images, followed by spatial features fed into a fully connected neural network for prediction (needing future surgery for uncontrolled glaucoma). The training process included mapping input data to the probability of requiring glaucoma surgery, followed by validation, performance evaluation, and iterative improvement.
Notably, our DLMs achieved AUC values over 0.8 at predicting future surgery from a single baseline ophthalmology visit. Our model also made surgical predictions for different time intervals, up to five years in the future – thus giving us a sense of how “urgent” the need for surgical intervention was. To do this, our DLM relied on several key input features: lower VF MD, higher IOP, thinner average OCT RNFL thickness, and higher VF PSD.
However, several considerations stand in the way of translating AI-driven predictive models from research settings to routine clinical practice. First, DLMs possess an inherent “black box” nature, which makes it difficult to interpret the underlying rationale behind their predictions. It is essential that clinicians understand how these models arrive at their predictions so that they can trust them for decision-making. Today, various techniques exist to generate and display model results interpretably to the clinician – such as feature importance in the results presented above.
In addition, DLMs heavily rely on large volumes of labelled data for training, and the quality, diversity, and representativeness of this data significantly impact model performance – biased or unrepresentative datasets can lead to skewed or unreliable predictions. Adhering to standards such as patient privacy and data security will, therefore, be essential for responsible model development and deployment.
For future work, prospective and external validation is essential; studies must prioritize assessing DLM performance across diverse patient populations and healthcare settings. Additionally, future work must seek to understand how patient outcomes change when AI-assisted care is implemented. Concurrently, we must optimize the deployment of DLMs in clinical settings by studying how best to integrate such models into the workflow and how best to leverage the complementary strengths of the AI model and the clinician to arrive at the best decision for our patients.
I predict that AI will become an integral component of glaucoma care within the next 10 years. However, we need to study the important details outlined above to ensure that AI’s potential is maximized.
References
- S Resnikoff, et al., “Estimated number of ophthalmologists worldwide (International Council of Ophthalmology update): Will we meet the needs?,” Br. J. Ophthalmol. 104, 4 (2020). PMCID: PMC7147181
- J Yohannan, et al., “Deep learning-based identification of eyes at risk for glaucoma surgery,” Sci Rep, 14, 1 (2024). 599. PMID: PMC10770345