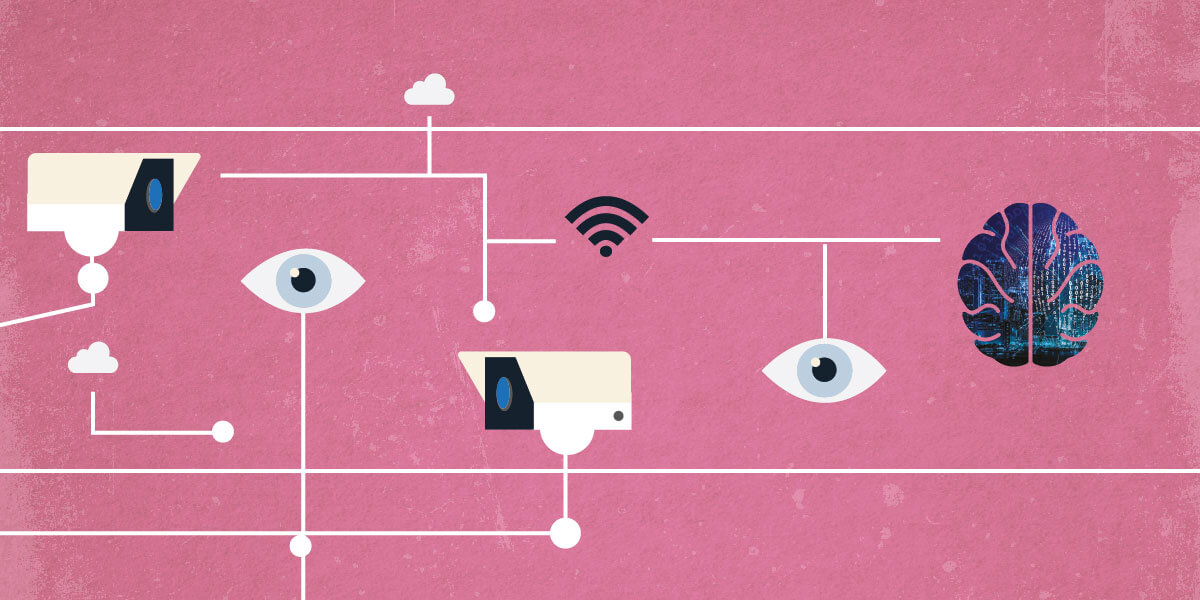
At A Glance
- Daniel Ting explains how AI is changing the medical imaging field, assisting in detection of medical conditions
- The deep learning system for retinal disease screening is currently being used to detect three conditions: glaucoma, referable diabetic retinopathy and AMD
- AI can be used for lifelong monitoring of a patient’s health and could track the progress of specific diseases
- In the future, AI could help predict the incidence and progression of various retinal conditions and even systemic diseases.
Many instances of ocular diseases go undiagnosed because of a lack of trained professionals to handle the vast amount of necessary screening. Algorithms capable of distinguishing referable images from those that don’t require extensive human scrutiny can help lighten the load – and could potentially be the answer to the challenge of overworked, under-resourced diagnostic specialists. Here, we gain the perspective of Daniel Ting, Assistant Professor at the Singapore National Eye Center, SingHealth Duke-NUS Medical School, National University of Singapore.
What developments have led to the rise of artificial intelligence in medicine?
AI has been around over the last few decades. Over the past few years, deep learning using graphic processing unit (GPU) servers has revolutionized the field of computer science, sparking tremendous interest in image recognition, speech recognition, and natural language processing. In medical imaging, deep learning has shown to be comparable, if not superior, to human graders in detection of many medical conditions, including diabetic retinopathy, skin melanoma, breast metastases and tuberculosis.
Tell us about the deep learning system you developed for retinal disease screening...
Our system is effective in using retinal images to automatically detect three conditions: referable diabetic retinopathy, glaucoma, and age-related macular degeneration. We are currently in the midst of developing algorithms for other retinal conditions, including retinal vein occlusions and retinal detachment.
The designs and concepts of AI algorithms are fairly similar across different medical disciplines. Most importantly, we need to know the right research questions to ask so that we can design the algorithm accordingly. Training datasets with labeled “ground truth” – that is, known results the computer can learn from – is equally (if not more) critical than the technical architecture of a deep learning system both within and outside ophthalmology. For instance, I am involved in several AI grants in radiology at Johns Hopkins University, looking at developing a chest radiograph algorithm to differentiate normal from abnormal X-rays and identify different lung diseases. Eventually, I hope to also apply AI to dermatology and tissue pathology.
What are the pros and cons of using AI as a diagnostic tool?
AI as a diagnostic tool has several obvious advantages, including cost and time savings, a sustainable workforce that will get smarter over time, and zero intra-rater variability. Our system in particular can reduce the total diagnostic workload by at least 50 to 70 percent, simply by removing the non-referable images so that the human graders can focus on the retinal images that need extra attention. AI is also useful for lifelong monitoring; once the machine has baseline patient data, it can easily compare future images to signal the progression – or resolution – of disease.
That’s not to say that there are no disadvantages to AI in the clinic. It requires a large dataset to train, for instance, which is not only time-consuming, but also creates a need for technical expertise and supporting infrastructure. The challenges are easing every day, as cloud-based services become cheaper and more readily available, but effort and expense will remain. In my opinion, the benefits of AI are certainly worth the process of establishing, training and maintaining it!
What lies ahead for your work – and for medical AI in general?
Our team has come a long way. My four co-inventors (Tien Wong, Wynne Hsu, Mong Li Lee and Gilbert Lim) and I began developing and testing this AI system five years ago using retinal images we collected over a decade ago. We’ve poured enormous financial and manpower resources into this project, and I’m thrilled that the system has overcome all of the initial obstacles and now shows promise in assisting diagnostic professionals (1).
AI is the fourth industrial revolution in human history. As far as I can see, it’s definitely set to revolutionize medicine over the next few decades. In ophthalmology, we hope that AI will help with the repetitive diagnostic workloads for diseases like diabetic retinopathy, glaucoma, and age-related macular degeneration. Using different retinal imaging modalities, AI can potentially help us see the changes to retinal neurovascular structures that have thus far been invisible to the human eye, and help us predict the incidence and progression of various retinal conditions and even systemic diseases. By having a robust algorithm, we also hope to deliver personalized medicine to the global population of patients with diabetes. There are similar trends in other medical specialties – not just ophthalmology and pathology, but also radiology, dermatology, oncology and others.
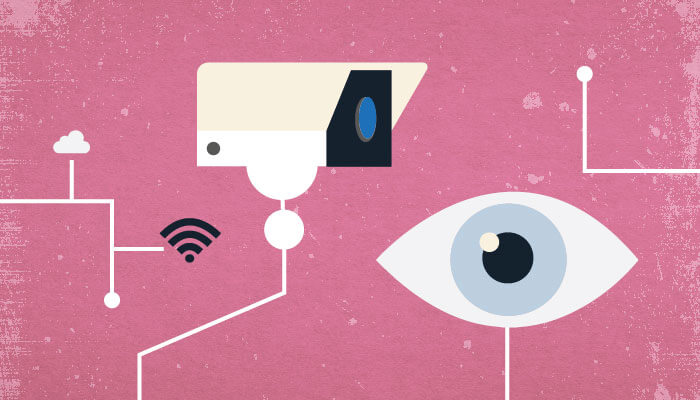
References
- 1. DSW Ting et al., “Development and validation of a deep learning system for diabetic retinopathy and related eye diseases using retinal images from multiethnic populations with diabetes”, JAMA, 318, 2211–2223 (2017). PMID: 29234807.