See It, Say It, Change It
If innovators are looking for far-reaching success, they must include data from all ethnicities
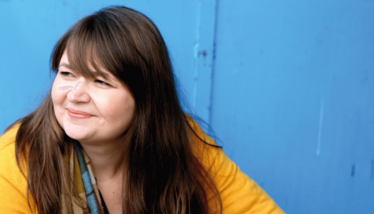
According to the gurus who shared their perspectives in this issue’s cover feature, system bias is a key challenge in the continuous development of AI in ophthalmology. As Michael Chiang, Director of the National Eye Institute at the NIH, describes it: “AI systems are typically trained and validated in fairly narrow populations and specific imaging devices, whereas real-world applications will need to be rigorously validated to ensure they work across broad populations and devices without bias.”
The same concern pops up time and time again. When I spoke with Anthony Khawaja, a Moorfields Eye Hospital glaucoma specialist and genomics expert, he was uncomfortable about making progress in medicine that would only benefit people from one ethnic background. “It seems clear that more work needs to be done to replicate prior research for other ethnic groups – and to develop a framework that leaves no group of patients disadvantaged” (1).
Although attempts are being made to deploy research projects in developing countries – for example, Zambian and UK ophthalmologists are using deep learning to screen for diabetic retinopathy (2) – most R&D is conducted in industrialized economies. Even in the most diverse populations, it is not always easy to gather data across different ethnicities, and, as Michael Abramoff told me previously (3): “There are legitimate concerns about racial and ethnic bias in AI. It is important that autonomous AI is designed and validated in a way that ensures those concerns are addressed, and any inappropriate bias is corrected.”
But that’s easier said than done when implicit bias is so prominent in society. Even electronic health records (EHR) have been perpetuating racial bias, as discovered by a group using machine learning techniques to analyze data of over 18,000 adult patients in Chicago (4). So, is there hope? The EHR project investigators found that the situation improved after March 1, 2020 – perhaps due to “social pressures [that] may have sensitized providers to racism and increased empathy for the experiences of racially minoritized communities.”
Visibility and awareness are key: you cannot change things you don’t, can’t, or won’t see. It is vital that we all shout from the rooftops about inequality – wherever we see it, including the sphere of ophthalmic innovation and research.
- A Jones, “Genomics and Glaucoma,” The Ophthalmologist (2019). Available at: https://bit.ly/3SLQoHU.
- V Bellemo et al., “Artificial intelligence using deep learning to screen for referable and vision-threatening diabetic retinopathy in Africa: a clinical validation study,” Lancet Digit Health, 1, e35 (2019). PMID: 33323239.
- A Jones, “The Ethics of AI,” The Ophthalmologist (2020). Available at: https://bit.ly/3UZT2vu.
- M Sun et al., “Negative patient descriptors: documenting racial bias in the Electronic Health Record,” 41, 203 (2022). PMID: 35044842.
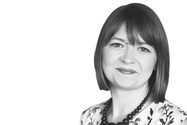
Having edited several technical publications over the last decade, I crossed paths with quite a few of Texere's current team members, and I only ever heard them sing the company's praises. When an opportunity arose to join Texere, I jumped at the chance! With a background in literature, I love the company's ethos of producing genuinely engaging content, and the fact that it is so well received by our readers makes it even more rewarding.