Dynamic Modeling of Ocular Disease Progression with Generative AI
Integrating the power of generative artificial intelligence into everyday clinical practice
Alfredo Di Giovanni | | 5 min read | Technology
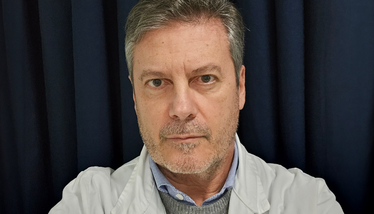
Alfredo Di Giovanni
The initial integration of artificial intelligence (Al) in ophthalmology focused on the automated classification of lesions and abnormalities in optical coherence tomography (OCT) images and fundus photographs, providing assistance in the early detection of diseases. Although these approaches proved effective, they essentially offered a static "snapshot" of the patient's condition at a given time. These static shots lacked the ability to predict the disease's evolution over time, and to assess the real-time influence of therapeutic strategies on its natural trajectory. In designing AI-based progression curves, I aimed to move beyond a static view of pathology, highlighting the disease’s natural evolution and the true impact of therapies.
Generative Al now allows us to overcome this limitation, transforming the logic from a point-in-time analysis to a dynamic mode. Predictive curves modeling the progression of chronic ocular conditions – conditions such as glaucoma, macular diseases, and progressive myopia – can now be created and dynamically reshaped to anticipate disease progression and understand how a therapeutic intervention might alter its course.
As opposed to the fixed snapshot of pathology we once had, we are now privy to a powerfully effective “movie” that allows us to watch in real-time as a disease evolves, offering physicians a direct comparison between the natural course of a disease and the impact of therapies taken by the patient.
Generative Al and predictive curves
Unlike traditional discriminative models, generative Al models do not merely classify existing data; they generate new scenarios based on learned patterns. In ophthalmology, this capability enables the creation of predictive curves for a disease, which can be updated at every new patient visit or after any therapeutic intervention. For example, in glaucoma, beyond estimating the risk of progression (e.g., RNFL thinning, visual field deterioration), it is now possible to have a baseline curve representing the disease's natural history and, by incorporating the latest data, observe to what extent a new therapy influences the expected trajectory.
In the case of macular diseases, such an approach allows for evaluation of the impact of intravitreal therapies on the evolution of morphological and functional alterations by comparing the observed situation with the predicted scenario. Similarly, in progressive myopia, predictions regarding axial length elongation and refractive worsening can be updated in light of preventive or therapeutic interventions, enabling an objective assessment of outcomes against a baseline framework.
Experience with OcuSmart
OcuSmart, a platform I developed that integrates the power of generative Al into everyday clinical practice, uses large language models and deep learning algorithms capable of processing complex data to offer a more informed and personalized decision-making support tool for ophthalmologists. OcuSmart's predictive modeling engine leverages cutting-edge large language model architectures – specifically fine-tuned instances of ChatGPT – to interpret and integrate multidimensional clinical datasets, enabling the generation of highly individualized disease trajectories.
Information from OCT exams, visual fields, refractive parameters, and intraocular pressure values are used to construct personalized predictive curves. Each parameter contributes to outlining a comprehensive disease profile, taking into account both the individual patient's characteristics and progression patterns learned from a large dataset of previous cases. The continuous updating of these curves occurs at each new patient visit: if a medication is introduced, a treatment changed, or a more stringent monitoring strategy adopted, subsequent data acquisition allows for a reshaping of the prediction. In this way, it becomes possible to quantify and visualize the impact of therapeutic decisions on the natural course of the disease, without delegating treatment choices to Al. The ophthalmologist remains the final decision-maker, interpreting the results in light of clinical experience and the patient's individual context.
Methods and data integration
To obtain reliable predictive curves, OcuSmart relies on generative Al algorithms trained on large and diverse datasets, including cases from different clinical settings. The quality of input data is paramount: high-resolution OCT scans, reliable visual fields, and accurate refractive and pressure parameters are essential to build trustworthy models. Moreover, the system adapts to individual patient differences, taking into account both known morphological and functional factors and variables, such as age, comorbidities, or specific anatomical features.
The algorithm employed is generative in nature (often based on deep neural networks such as Transformer models), capable of learning data distributions and producing plausible future scenarios. This capability not only allows for extrapolation of trends but also for estimating the probability of significant changes in clinical parameters, providing a quantitative basis for assessing therapeutic efficacy.
The Ophthalmologist Presents:
Enjoying yourself? There's plenty more where that came from! Our weekly newsletter from The Ophthalmologist brings you the most popular stories as they unfold, chosen by our fantastic Editorial team!
Benefits in clinical practice and safety considerations
This approach makes it possible to move from a strategy based on statistical evaluations and empirical attempts to a more systematic one supported by quantitative indicators. If a therapy slows glaucoma progression, the updated predictive curve will show the difference compared to the initially expected trajectory. If no changes are observed, it becomes easier to consider alternative strategies in a timely manner.
Using dynamic predictive curves also leads to greater patient awareness: clearly demonstrating how the disease could progress without intervention and how it is currently evolving under the ongoing therapy can improve understanding and treatment adherence.
The integration of generative Al into clinical practice requires special attention to data protection and anonymity. As such, OcuSmart implements strict cybersecurity and privacy protocols. Furthermore, it is crucial that clinicians maintain an approach in which generative Al provides decision support, rather than serving as a substitute for medical judgment.
Future perspectives
Integrating multimodal data – such as biomarkers, genetic parameters, and lifestyle information – could further enhance the predictive capabilities of generative Al. This may foster increasingly personalized therapeutic approaches and offer more robust decision support, even in settings with limited resources, thus contributing to greater equity in healthcare access.
The use of generative Al in ophthalmology demonstrates the feasibility of moving from static data analysis to a predictive and dynamic model. The ability to create and reshape predictive curves at each visit provides a more objective assessment of how therapies impact the natural course of chronic ocular diseases. Without replacing the clinician's expertise, this approach enhances decision-making capabilities, contributing to more informed and targeted management of ocular diseases in a continuously evolving landscape.
Dr. Alfredo Di Giovanni has taught for many years at the School of Specialization in Ophthalmology at the Faculty of Medicine and Surgery of the University of Ferrara (Italy). He currently works at ASL Napoli2Nord and is particularly involved in new technologies and artificial intelligence applied to Ophthalmology.