AI: The Future No Longer
“How will AI impact ophthalmology?” There’s no time left to answer.
Julian Upton | | 5 min read | Opinion
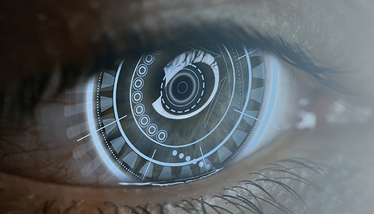
Credit: Shutterstock.com
“Artificial intelligence (AI) is not just something out of a science fiction movie… It’s a technology of today that surrounds us wherever we go.” So wrote Rahul N. Khurana on the American Academy of Ophthalmology (AAO) website in July 2018 (1). Khurana went on to identify two ways AI is changing the practice of ophthalmology. The first – improving screening and access to care – saw ophthalmologists “using AI to screen fundus images automatically for conditions like diabetic retinopathy and glaucoma,” wrote Khurana. The second, augmenting intelligence, addressed the information/data “flood” facing the modern-day ophthalmologist. “AI will be the backbone to help us manage this information overload and deliver the optimal care for our patients.”
Of course, the subject of AI in ophthalmology – and, certainly, in healthcare more broadly – has been a popular thread at conferences for a decade or more. Perhaps what is more concerning is the question – “What is the future of AI in ophthalmology?” – remains ubiquitous. Given the advances made in the last five years since Khurana’s prediction alone – maybe we should be asking, “How do we use AI now?”
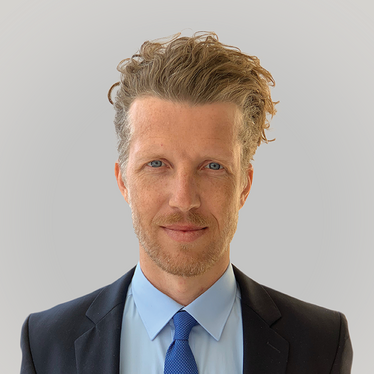
Josef Huemer, Consultant Ophthalmologist for Medical Retina at Moorfields Eye Hospital NHS Foundation Trust. (Heashot supplied by Josef Huemer.)
Or perhaps: “Why now?”
For that was the question posed by Josef Huemer, Consultant Ophthalmologist for Medical Retina at Moorfields Eye Hospital NHS Foundation Trust, London, at this year’s European Society of Cataract and Refractive Surgeons (ESCRS) meeting in Vienna, Austria (September 8–12, 2023). According to Huemer, the last 10 years have shown how deep learning has changed image analysis and speech recognition, and we have just entered the epoch of generative AI. Every OCT scan provides 65 million data points – and we have large data sets of fundus images for diseases like diabetic retinopathy (DR), says Huemer. Since 2000 there have been more than 3000 publications focused on AI in ophthalmology. And the technology has provided FDA-approved applications for retinal diseases, such as diabetic retinopathy (DR). Moreover, it also has shown promising results in intraocular lens positioning, confocal microscopy, cataract grading, capsular bag diameter prediction, and diagnosing keratoconus, to name a few. Huemer adds, “Just recently, a group of researchers including Pearse Keane of Moorfields Eye Hospital and UCL Institute of Ophthalmology published a widely praised article on the first foundation model in ophthalmology, and much progress has been made in finding signs of Parkinson’s disease on OCT scans – potentially opening up new use cases in the coming years” (2).
“We have the proof of concept,” Huemer tells The Ophthalmologist. “The interesting bit now – the difficult part – is how do we make the best use of our data sets? How do we ensure they are representative? How can we translate what we have learnt from, say, DR and other diseases, with clinical safety?”
A pressing concern, certainly, is the danger of bias and how AI can enhance and exaggerate that bias when it comes to dealing with diverse populations. “This is something we still don’t know enough about,” says Huemer. That AI can be “racist” – or, at least, be able to detect race without being trained to do so – is “a scary thing.” We need to have a regulatory framework in place and “get to a mindset where we can address this problem,” explains Huemer. He points to the work led by Alastair Denniston, Consultant Ophthalmologist and Professor at the Institute of Inflammation and Ageing at the University of Birmingham, amongst other positions, whose work on the international STANDING Together collaboration aims to “develop best-practice standards for healthcare datasets used in AI, ensuring they are diverse, inclusive, and don’t leave underrepresented or minoritized groups behind” (3).
Deep-learning systems, says Huemer, must be subject to “robust validation processes in multi-ethnic cohorts to avoid biases.” One promising example is the UK DR screening program, which includes a multi-ethnic population. “This could serve to see if AI works equally well for everybody – and does exactly what we want it to do,” says Huemer. As he has previously noted: “There are indications that we may yet be able to democratize the power of effective DR screening on a global scale” (4).
On that other ever-present (if more provocative) question – “Will AI replace doctors?” – Huemer is less concerned. There are cases where such a development will be useful, he says, triaging patients with AI, for example, or performing routine tasks that are time consuming and tedious for humans. “But there are other areas of ophthalmology where I don’t think AI will ever be that useful,” he adds. A subspecialty such as uveitis is a good example of this – mainly because it’s a heterogeneous field with small case numbers; “A doctor with great clinical expertise will always be better in this instance.” However, Huemer concludes, “Let’s not see AI as a threat, but rather as an opportunity. Remember, doctors were not keen when the thermometer was introduced to measure body temperature, and that has not replaced us. Nor has the use of OCTs replaced retina specialists.”
- Rahul N. Khurana, “2 Ways AI Is Changing the Practice of Ophthalmology,” AAO.org, July 2015. bit.ly/3ulcRoq
- Yukun Zhou et al., “A foundation model for generalizable disease detection from retinal images,” Nature, 622, 156 (2023). DOI: 10.1038/s41586-023-06555-x
- University of Birmingham, “Public support needed to tackle racial and other biases in AI for healthcare,” (September 26, 2022). bit.ly/3QMrQPO
- J Huemer et al., “The Evolution of Diabetic Retinopathy Screening Programmes: A Chronology of Retinal Photography from 35 mm Slides to Artificial Intelligence,” Clin Ophthalmol., 14, 2021 (2020). PMID: 32764868.
Julian Upton is Group Editor of The Ophthalmologist and The New Optometrist. With 20+ years' experience of the magazine industry, he has covered many facets of science and healthcare.