AI: Shaping the Future of Medicine
How AI-integrated retinal imaging is revolutionizing contemporary eye care
Andrzej Grzybowski, Kai Jin | | 3 min read | Future

Credit: The Ophthalmologist

Andrzej Grzybowski (Headshot supplied by author)

Kai Jin (Headshot supplied by author)
The integration of artificial intelligence (AI) with multimodal data analysis, including eye imaging, presents a revolutionary approach to not only diagnosing eye diseases, but also predicting systemic conditions affecting various body systems (1), including the brain and cardiovascular system (2) (see Figure 1). This radical change in imaging approaches has the potential to redefine medical diagnostics and enhance our understanding of the interplay between age, sex, and race in disease pathology.
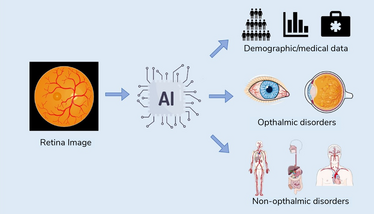
Figure 1. Overview of the applications of AI algorithms based on retinal photos.
In contemporary ophthalmology, the fusion of data from diverse sources, such as optical coherence tomography (OCT), fundus photography, and genetic profiling, allows for a comprehensive evaluation of ocular health (3). AI-driven algorithms can process these multimodal datasets to detect early signs of ocular diseases, such as glaucoma, age-related macular degeneration (AMD), and diabetic retinopathy (4), aiding not only early disease diagnosis, but also helping track disease progression.
Beyond eye-specific conditions, the integration of multimodal data holds immense potential for predicting other systemic diseases. For instance, in the case of neurological diseases, AI analysis of ocular data can help to identify subtle changes in the retina associated with Alzheimer’s disease (5), Parkinson’s disease (6), and multiple sclerosis, facilitating early diagnosis and monitoring of these conditions, and potentially paving the way for personalized treatment strategies. Alongside genetic and clinical data, retinal imaging can also contribute to the prediction of cardiovascular diseases (7), such as hypertension and atherosclerosis. To assess cardiovascular risk, AI analyzes retinal vessel caliber and morphology, enabling timely interventions to prevent heart disease and stroke.
The incorporation of demographic data into AI-based ophthalmic analysis is also a burgeoning field. Advanced AI algorithms can quantify age-related changes in the eye, including lens opacity, retinal thickness alterations, and macular degeneration (8); understanding these changes in the context of aging is critical for accurate diagnostics and treatment planning. Recent research is also being conducted into exploring sex-based differences in eye anatomy and disease susceptibility (9), with studies suggesting that women may be more prone to certain eye conditions like dry eye syndrome (10), and AI can aid in deciphering the nuances of these sex-related disparities in ocular health.
In terms of AI-applied race recognition in medical imaging, we can say it is an evolving frontier. Recognizing any racial disparities in disease prevalence and outcomes can be essential for addressing healthcare inequities (11), and AI can contribute to this field by analyzing medical images for race recognition and subsequently investigating the impact of race on disease susceptibility and progression. Incorporating gathered race-specific data into diagnostic and treatment algorithms can also enable more tailored and equitable healthcare delivery, accounting for genetic and environmental factors that vary across populations.
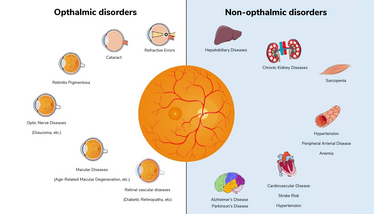
Figure 2. The application of retinal photo-based AI in both ophthalmic and non-ophthalmic conditions.
The integration of AI with multimodal data analysis in ophthalmology represents a paradigm shift in healthcare. It offers the potential to not only diagnose eye diseases more accurately, but also to predict and manage systemic conditions affecting various organ systems (see Figure 2). Moreover, the analysis of age, sex, and race-related factors further enhances our understanding of disease pathology and healthcare disparities. As AI continues to advance, the synergy between ophthalmology and AI-driven analysis will undoubtedly play a pivotal role in shaping the future of medicine, offering personalized, data-driven, and equitable healthcare solutions for all.
- TH Rim et al., “Prediction of systemic biomarkers from retinal photographs: development and validation of deep-learning algorithms,” The Lancet Digital health, 10, 526 (2020). PMID: 33328047.
- K Sathianvichitr et al., “Through the eyes into the brain, using artificial intelligence,” Annals of the Academy of Medicine, 2, 88 (2023). PMID: 36880820.
- U Schmidt-Erfurth et al., “Artificial intelligence in retina,” Progress in retinal and eye research, 67, 1 (2018). PMID: 30076935.
- K Jin, J Ye, “Artificial intelligence and deep learning in ophthalmology: Current status and future perspectives,” Advances in Ophthalmology Practice and Research. (2022).
- CY Cheung et al., “A deep learning model for detection of Alzheimer’s disease based on retinal photographs: a retrospective, multicentre case-control study,” The Lancet Digital health, 11, 806 (2022). PMID: 36192349.
- W Hu et al., “Retinal age gap as a predictive biomarker of future risk of Parkinson’s disease,” Age and aging, 51, 3 (2022). PMID: 35352798.
- L Arnould et al., “Using Artificial Intelligence to Analyze the Retinal Vascular Network: The Future of Cardiovascular Risk Assessment Based on Oculomics? A Narrative Review,” Ophthalmology and therapy, 2, 657 (2023). PMID: 36562928.
- Y Peng et al., “DeepSeeNet: A Deep Learning Model for Automated Classification of Patient-based Age-related Macular Degeneration Severity from Color Fundus Photographs,” Ophthalmology, 4, 565 (2019). PMID: 30471319.
- R Poplin et al., “Prediction of cardiovascular risk factors from retinal fundus photographs via deep learning,” Nature biomedical engineering, 3, 158 (2018). PMID: 31015713.
- C Matossian et al., “Dry Eye Disease: Consideration for Women’s Health,” J Womens Health (Larchmt), 4, 502 (2019). PMID: 30694724.
- AS Coyner et al., “Association of Biomarker-Based Artificial Intelligence With Risk of Racial Bias in Retinal Images,” JAMA ophthalmology, 6, 543 (2023). PMID: 37140902.
Andrzej Grzybowski is a professor of ophthalmology at the University of Warmia and Mazury, Olsztyn, Poland, and the Head of Institute for Research in Ophthalmology at the Foundation for Ophthalmology Development, Poznan, Poland. He is EVER President, Treasurer of the European Academy of Ophthalmology, and a member of the Academia Europea. He is a member of the International AI in Ophthalmology Society (https://iaisoc.com/) and has written a book on the subject that can be found here: link.springer.com/book/10.1007/978-3-030-78601-4.
Kai Jin is a doctor and research scientist at the Eye Center, the Second Affiliated hospital of Zhejiang University School of Medicine. His current research interests are in retina, imaging, artificial intelligence, and personalized medicine. He received his PhD in 2018 and has an outstanding publication record, with 54 publications in peer-reviewed international journals. Jin’s Scopus Scientific Citations number is 1092 and his Hirsch index is 18. In the past year, Jin was awarded the WILEY Top Cited Article Award and the National Scholarship.